Thanks to Wil Perkins, Grant Fleming, Thomas Larsen, Declan Nishiyama, and Frank McBride for feedback on this post. Thanks also to Paul Christiano, Daniel Kokotajlo, and Aaron Scher for comments on the original post that helped clarify the argument. Any mistakes are my own.
In order to submit this to the Open Philanthropy AI Worldview Contest, I’m combining this with the previous post in the sequence and making significant updates. I'm leaving the previous post, because there is important discussion in the comments, and a few things that I ended up leaving out of the final version that may be valuable.
Introduction
In this post, I argue that deceptive alignment is less than 1% likely to emerge for transformative AI (TAI) by default. Deceptive alignment is the concept of a proxy-aligned model becoming situationally aware and acting cooperatively in training so it can escape oversight later and defect to pursue its proxy goals. There are other ways an AI agent could become manipulative, possibly due to biases in oversight and training data. Such models could become dangerous by optimizing directly for reward and exploiting hacks for increasing reward that are not in line with human values, or something similar. To avoid confusion, I will refer to these alternative manipulative models as direct reward optimizers. Direct reward optimizers are outside of the scope of this post.
Summary
In this post, I discuss four precursors of deceptive alignment, which I will refer to in this post as foundational properties. I first argue that two of these are unlikely to appear during pre-training. I then argue that the order in which these foundational properties develop is crucial for estimating the likelihood that deceptive alignment will emerge for prosaic transformative AI (TAI) in fine-tuning, and that the dangerous orderings are unlikely. In particular:
- Long-term goals and situational awareness are very unlikely in pre-training.
- Deceptive alignment is very unlikely if the model understands the base goal before it becomes significantly goal directed.
- Deceptive alignment is very unlikely if the model understands the base goal significantly before it develops long-term, cross-episode goals.
Pre-training and prompt engineering should enable an understanding of the base goal without developing long-term goals or situational awareness. On the other hand, long-term goals and will be much harder to train.
Definition
In this post, I use the term “differential adversarial examples” to refer to adversarial examples in which a non-deceptive model will perform differently depending on whether it is aligned or proxy aligned. The deceptive alignment story assumes that differential adversarial examples exist. The model knows it’s being trained to do something out of line with its goals during training and plays along temporarily so it can defect later. That implies that differential adversarial examples exist in training.
Implications of this argument
Many existential AI catastrophe scenarios rely heavily on deceptive alignment. So, if deceptive alignment is highly unlikely (<1%) to emerge for TAI, we should update our expectations for AI existential risk accordingly. This conclusion also warrants a re-evaluation of priorities for alignment research areas and between cause areas. Other possible alignment research topics include governance, direct reward optimizers, and multipolar scenarios that don’t rely on deceptive alignment.
Assumptions about the TAI training paradigm
I’m assuming prosaic development of TAI, using a training process like human feedback on diverse tasks (HFDT). The goal of the training process would be a model that follows directions subject to non-consequentialist ethical considerations. This high-level training setup is already the default training process for text models such as GPT-4, and this will likely continue because of the flexibility and strong performance it provides. I also expect unsupervised pre-training to be an important part of TAI development. You can find justifications for these assumptions here.
Foundational properties for deceptive alignment
There are four foundational properties that are key to the deceptive alignment story. These foundational properties are:
- Goal-directed behavior - the model needs to have some goal or set of goals about the external world.
- Optimizing across episodes/long-term goal horizons - model behavior needs to be influenced by long-term goals.
- Conceptualization of the base goal - the model needs to understand what the base goal is in order to successfully play along in training.
- Situational awareness - the model needs to understand that it is a model in training. Furthermore, it needs to understand how its actions now could affect its parameter updates, and therefore future goal attainment.
The order in which these develop, and the amount of training that goes on in between the development of foundational properties, will have a large effect on the likelihood of deceptive alignment.
Pre-trained models are unlikely to develop the necessary properties
Pre-trained models are unlikely to have long-term goals
In a typical pre-training process, a model's predicted output for token n is not used in the calculation or scoring of token n+1, and thus loss is calculated independently. There's not an incentive for gradient descent to learn sacrifice performance on the current word for performance on a future word in pre-training.
Furthermore, if a model gained marginally longer-term goals, but not long-term enough to enable deceptive alignment, the longer-term goals would be a competing priority and harm immediate reward in expectation. Gradient descent should therefore push against this.
The deceptive alignment literature argues that gradient descent could modify a model's goals to be long-term, because that would make it more deceptive and therefore increase training reward. There are therefore some concerns about long-term goals and deceptive alignment in pre-training. However, this is unlikely. For the sake of argument, suppose we have a model in pre-training that has a misaligned proxy goal and relevant situational awareness. But so far, it does not have a long-term goal.
In order to be deceptively aligned, the model has to have a long enough goal horizon to value its total goal achievement after escaping oversight more than its total goal achievement before escaping oversight. But pre-training processes are incredibly long (~the whole internet for text models). A situationally aware model should anticipate a lot of subsequent pre-training, and that the oversight will likely remain strong for a significant portion of fine-tuning. That requires a very long-term goal for any amount of deceptive alignment to be worthwhile.
Gradient descent updates parameters in the direction that most improves performance hyper-locally. Under the conditions I’ve described, making a myopic model's goals infinitesimally longer-term would not make deceptive alignment worthwhile and would harm training reward. Therefore, I don’t expect the gradients to point toward longer-term goals.
Pre-trained models unlikely to develop situational awareness
It's hard to imagine how a model would develop situational awareness in pre-training when:
- Situational awareness only produces deceptive alignment if the model already has long-term goals, and vice versa. Gradient descent is based on partial derivatives, which are independent of each other, so:
- If the model doesn't already have long enough goal horizons for deceptive alignment, then marginally more situational awareness doesn't increase deceptive alignment.
- If the model doesn't already have the kind of situational awareness necessary for deceptive alignment, then a marginally longer-term goal doesn't increase deceptive alignment.
- Therefore, the partial derivatives shouldn't point toward either property unless the model already has the other.
- Unlike in fine-tuning, the vast majority of internet text prompts do not contain information about situational context for the model to figure out that it is an ML model. Understanding that it is a model in training is not relevant for predicting next tokens on the internet, so there's no benefit to learning this information.
Dangerous foundational property development order is unlikely in fine-tuning
How complete does the understanding of the base goal need to be?
My central argument for fine-tuning is about when an understanding of the base goal emerges relative to other properties. But how accurate and specific does that understanding have to be to avoid deceptive alignment?
The relevant factor is whether the model’s internal goal is its own understanding of the base goal, even if that understanding is flawed. Suppose for the sake of argument that we have a model with an exceptionally long-term goal and situational awareness. Its internal goal is its best, but still flawed, representation (X) of the base goal (X`). This model can’t tell the difference between the base goal and the internal proxy goal, so it can’t be deceptively aligned yet. In short, it thinks it is aligned. If it performs worse than it could have been on a training example because of this, the gradients should point toward a stronger concept of the base goal, and therefore stronger alignment.
For example, let’s say we are training this model to follow directions from the prompt, unless they violate ethical norms (X`). The model understands that the base goal is to follow directions subject to some ethical norms but has an understanding of these norms (X) that is incorrect in some situations. Because it doesn’t understand the difference, it can’t act aligned for instrumental purposes, and it will get suboptimal reward when flaws in its understanding of the base goal affect its behavior. Because it is already pointing its goal at this flawed concept, the gradients should point toward improving the internal goal (X) to better match the base goal (X`). In order to become deceptively aligned, it has to have separate concepts for its internal goal and the base goal.
Now consider an alternate version of the above example, where the model has the same flawed concept for the base goal, but its internal goal is maximizing paperclips (or anything else that’s represented by a separate concept from its base goal representation). This model can immediately become deceptively aligned, because optimizing for its flawed understanding of the base goal will get better training performance than optimizing for paperclips in the short run. The model can then continue learning about the base goal without systematically changing its internal goals and remain deceptively aligned.
Will understanding of the base goal come before goal-directed behavior?
Why does this matter?
A key idea of deceptive alignment is early in training, a model does not understand the base goal, so it starts by learning a proxy that becomes its internal goal. But if the model understands the base goal before it develops a mesa-objective, then assuming differential adversarial training, there will be an incentive to optimize for the base goal, rather than a proxy. If the understanding of the base goal has already been calculated, there will be no reason to create and maintain a separate proxy goal when the model could just point at the base goal.
If conceptualization of the base goal and goal-directed behavior emerge at essentially the same time, deceptive alignment also seems unlikely. If a model converged on a solid representation of the base goal, and became goal-directed in parallel, then differential adversarial examples would provide an incentive for gradient descent to optimize for the base goal.
The most dangerous case is when the model develops a proxy objective before it understands the base goal.
I’ve seen some arguments that rely on the idea that deceptive alignment will not occur in the parameters, but the activations. This argument doesn't change the conclusion from this section. If the model figured out what you wanted it to do in the activations before it became significantly goal-directed, gradients could point towards parameters that accomplish those objectives after they have been inferred.
How likely is the dangerous case?
It is highly likely that unsupervised pre-training will be the first step in the TAI training process. Models that are only pre-trained almost certainly don’t have dangerous goals. They might have extremely myopic goals like next-token prediction, but those goals will necessarily start to shift when the model moves to fine-tuning. However, because a pre-trained model will already have high-level representations of key base goal concepts such as direction following and non-consequentialist ethical considerations, all it will have to do to become aligned is to point them. These models also understand language, and we can tell them what we want them to do from the start of fine-tuning. This kind of prompt engineering is already standard practice, and it's hard to imagine why we wouldn't use it going forward.
Differential adversarial examples should provide a strong incentive for gradient descent to point at the base goal, rather than a proxy. The deceptive alignment story assumes that differential adversarial examples exist. The model is explicitly changing its behavior to avoid correction, meaning that its internal goals produce different results from the base goal on training examples. If they did not exist, that would imply that a perfect or near-perfect proxy exists on a diverse training set. It's hard to imagine what that proxy could be if the goal is to follow directions subject to some constraints in diverse situations.
The argument presented in this section only applies to domains where large amounts of pre-training data exist. For example, AI operating robotics may not work this way. However, if researchers were able to produce a robustly aligned model for domains where pre-training is available, such as text and video generation, that would likely make it a lot easier to align robotics. The aligned, non-embodied AI could help with alignment research. Also, general purpose systems that include robotics, such as a scaled-up version of Gato, will be easier to align if we can robustly align key component parts such as text modeling. Alignment of the language portion of a general agent would also enable prompt engineering to help with overall alignment.
Will understanding of the base goal come before long-term goal horizons?
Why does this matter?
If a model has long-term goal horizons, then it must be goal-directed. However, it’s possible for the model to develop foundational properties in the following order:
- Goal-directed behavior
- Conceptualization of the base goal
- Optimizing across episodes/long-term goal horizons
If this happens, there will be a period of time when the model wants to optimize for a flawed proxy and understands the base goal but does not yet have the capabilities necessary for deceptive alignment. Because the model is not yet deceptively aligned, differential adversarial examples in this part of training should directly modify the model’s internal objective. If this period lasts for a significant number of differential adversarial examples, the model should be able to update its goals to point at its conception of the base objective.
The most dangerous case, which underpins the arguments described in the deceptive alignment literature, is if long-term, cross-episode goals develop before or around the same time as an understanding of the base goal.
How likely is the dangerous case?
A model can’t become deceptively aligned unless it has long-term goals that extend beyond the current training episode. It’s hard to see how gradient descent could train this cross-episode goals, especially if cross-episode goals are not a specific training objective. Gradient descent does not have a long-term plan for how it will achieve high reward. All it does is update parameters in the direction that would most improve its performance on the current training batch, hyper-locally. It can’t systematically optimize for anything else.
Therefore, it’s hard to imagine why gradient descent would incentivize sacrificing performance in the current training batch to achieve future gains in episodes that are not included in the training calculations. If you rewarded a model for things that happened in a different episode, then that would actually just expand the current episode, so it likely wouldn't generalize further than that. There may be ways to train cross-episode optimization, but it’s hard to imagine it happening early in training by accident. Similarly, it is probably difficult to train long goal horizons within an episode without explicitly rewarding a model for activities that occurred long before the reward.
Therefore, to deliberately train a model to have very long-term goals, you would likely need to train it over at least the duration of the intended goal. Deceptive alignment would likely require very long-term goals if it were early enough in training that the model did not have a concept of the base goal. If the duration of the goal were years or decades, then training would likely require years or decades of training data, even with very sophisticated training techniques. Even then, predicting the effects of your actions over long time frames in complex environments is hard, even for highly intelligent entities. Credit assignment would be quite difficult. This should provide plenty of time for the model to point at the right goal before it can become deceptively aligned.
I expect ML researchers to deliberately train models to have long-term goals. However, I expect researchers to start by reinforcing a pre-trained model on shorter-term goals. This approach would almost certainly be a lot easier and create a good foundation to build on. In contrast, understanding the base goal will be an important training priority immediately.
Some have proposed that ML models could learn to have long-term goals because that would enable deceptive alignment and therefore high training performance, but this argument falls flat in fine-tuning for the same reasons as in pre-training.
Skepticism of empirical examples of deceptive alignment
There are a lot of people in the alignment community who have studied and built a wide range of deep learning models in detail. But the only cases of internally misaligned machine learning models I’ve seen are toy cases that deliberately create this effect by creating a perfect proxy for the objective in the training set. In contrast, the deceptive alignment story assumes that the model's proxies are not perfect proxies in training. Otherwise, what would the instrumental incentive for alignment be? I don’t think these toy examples say anything about whether large, complex models will become deceptively aligned by accident. It's not surprising that you can intentionally create internal misalignment.
In contrast, I suspect that this community has put a lot less effort into simulating evolution. But the only non-contrived example of internal misalignment happening by accident that I’m aware of is from a simulation of evolution!
Gene propagation was the “reward” in this example. The goal of filtering high-reproduction organisms in training was to control for reproduction rate and isolate for other factors for gene survival. The analogy for a misaligned goal is reproducing at a high rate anyway. However, in this case, the simulation moves back and forth between a training process that discourages high reproduction rates and a deployment environment that encourages high reproduction rates. There is optimization in both settings, but in opposite directions. Therefore, it’s not surprising that evolution would find a way to act deceptively. Avoiding optimization in different phases of training versus deployment is a key strategy for making sure deceptive alignment doesn’t happen. Let’s look at some possible high-level training/testing regimes for TAI:
- One long training process, followed by deployment in which there are no parameter updates - here, there is no optimization power from the testing process, so I would not expect deceptive alignment.
- Iteratively move between training, in which updates are made, and deployment, in which updates are not made - here, you have the property where you move back and forth between training and deployment, but you do not have any optimization power in the deployment phase. Crucially, you could start with a fresh prompt in training and the model would have no way to recall information from deployment.
- A training process in which updates are made, and deployment, in which parameter updates are made based on things that happen in the wild. In this case, you do have optimization power in both training and deployment, but the optimization power would be in the same direction (towards what you want). Therefore, I would not expect the type of dangerous optimization seen in this evolution experiment. Even if you continued iterating between training and this mixed version of deployment, you would be training it in roughly the same way on both. In practice, the training/deployment distinction might get blurry, but that should be fine.
None of these produce incentives that point in different directions for training versus deployment.
Implications for overall AI x-risk
Deceptive alignment is central to many descriptions of how transformative AI could cause an existential risk. If it is unlikely, then we should update our estimates of risk accordingly. Other AI failure modes include direct reward optimizers and some multipolar scenarios that don’t rely on deceptive alignment. If deceptive alignment is very unlikely for TAI, then research on alternative governance and misalignment scenarios should take precedence over deceptive alignment. It’s also worth re-evaluating how high of a priority AI risk should be. This would represent a serious shift from the status quo. As a side benefit, deceptive alignment is also the main line of argument that sounds like science fiction to people outside of the alignment community. Shifting away from it should make it easier to communicate with people outside of the community.
Conclusion
The standard deceptive alignment argument relies on foundational properties developing in a very specific order. However, this ordering is unlikely for prosaic AI. Long-term goals and situational awareness are not realistic outcomes in pre-training. In fine-tuning, it's very unlikely that a model would develop a misaligned long-term goal before its goal became aligned with the training goal. Based on this analysis, deceptive alignment is less than 1% likely for prosaic TAI. This renders many of the doom scenarios that are discussed in the alignment community unlikely. If the arguments in this post hold up to scrutiny, we should redirect effort to governance, multipolar risk, direct reward optimizers, and other cause areas.
Appendix
Justification for key assumptions
I have made 3 key assumptions in this post:
- TAI will come from prosaic AI training.
- TAI will involve substantial unsupervised pre-training.
- TAI will come at least in part from human feedback on diverse tasks.
I justify them in this section.
TAI will come from prosaic AI
It’s possible that there will be a massive paradigm shift away from machine learning, and that would negate most of the arguments from this post. However, I think that this shift is very unlikely. Historically, attempts to create powerful AI without machine learning have been very disappointing. Given the success of ML and the amount of complexity that seems necessary even for narrow intelligence, it would be quite surprising for TAI to emerge without machine learning. Even if it did, the order of foundational properties development would still matter, as described in my previous post.
The arguments in this post don’t rely on any particular machine learning architecture, so the conclusions should be robust to different architectures. It’s possible that gradient descent will be replaced by something that doesn’t rely on gradients and local optimization, which would undermine some of these arguments. This possibility also doesn’t seem likely to me, given the difficulty of optimizing trillions of parameters without taking small, local steps. As far as I can tell, the alignment community largely shares this belief.
TAI will involve substantial unsupervised pre-training
Pre-training already enables our AI to model human language effectively. It leverages massive amounts of data and works very well. It would be surprising for someone to try to develop TAI without using this resource. General-purpose systems could easily incorporate this, and it would take something extreme to make that obsolete. Human language is complicated, and it’s hard to imagine modeling that from scratch without a large amount of data.
TAI will come at least in part from human feedback on diverse tasks
This post assumes that the goal of training is a general, direction following agent using human feedback on diverse tasks. However, the most likely alternative training regimes don’t change the conclusions. For example, if TAI instead came from training a model to automate scientific research, the model would presumably still include a significant pre-trained language component. Furthermore, scientific research involves a lot of thorny ethical questions. There also needs to be a way to tell it what to do, and direction following is a straightforward solution for that. Therefore, there is a strong incentive to train non-consequentialist ethical considerations and direction following as the core functions of the model, even though its main purpose is scientific research. This approach provides a lot of flexibility and will likely be used by default.
There are also some possible augmentations to the human feedback process. For example, Constitutional AI uses reinforcement learning from human feedback (RLHF) to train a helpful model, then uses AI feedback and a set of principles to train harmlessness. This kind of implementation detail shouldn’t significantly affect foundational property development order, and therefore would not change my conclusion.
If a model is deceptively aligned after fine-tuning, it seems most likely to me that it's because it was deceptively aligned during pre-training.
"Predict tokens well" and "Predict fine-tuning tokens well" seem like very similar inner objectives, so if you get the first one it seems like it will move quickly to the second one. Moving to the instrumental reasoning to do well at fine-tuning time seems radically harder. And generally it's quite hard for me to see real stories about why deceptive alignment would be significantly more likely at the second step than the first.
(I haven't read your whole post yet, but I may share many of your objections to deceptive alignment first emerging during fine-tuning.)
I've gotten the vague vibe that people expect deceptive alignment to emerge during fine-tuning (and perhaps especially RL fine-tuning?) but I don't fully understand the alternative view. I think that "deceptively aligned during pre-training" is closer to e.g. Eliezer's historical views.
Paul, I think deceptive alignment (or other spontaneous, stable-across-situations goal pursuit) after just pretraining is very unlikely. I am happy to take bets if you're interested. If so, email me (alex@turntrout.com), since I don't check this very much.
I agree, and the actual published arguments for deceptive alignment I've seen don't depend on any difference between pretraining and finetuning, so they can't only apply to one. (People have tried to claim to me, unsurprisingly, that the arguments haven't historically focused on pretraining.)
Doesn't deceptive alignment require long-term goals? Why would a model develop long-term goals in pre-training?
Because they lead to good performance on the pre-training objective (via deceptive alignment). I think a similarly big leap is needed to develop deceptive alignment during fine-tuning (rather than optimization directly for the loss). In both cases the deceptively aligned behavior is not cognitively similar to the intended behavior, but is plausibly simpler (with similar simplicity gaps in each case).
For the sake of argument, suppose we have a model in pre-training that has a misaligned proxy goal and relevant situational awareness. But so far, it does not have a long-term goal. I'm picking these parameters because they seem most likely to create a long-term goal from scratch in the way you describe.
In order to be deceptively aligned, the model has to have a long enough goal horizon so it can value its total goal achievement after escaping oversight more than its total goal achievement before escaping oversight. But pre-training processes are incredibly long (~the whole internet for text models). A situationally aware model should anticipate a lot of subsequent pre-training, and that the oversight will likely remain strong for many iterations after pre-training. That requires a very long-term goal for any amount of deceptive alignment to be worthwhile.
Gradient descent updates parameters in the direction that most improves performance hyper-locally. Under the conditions I’ve described, making goals infinitesimally longer-term would not make deceptive alignment worthwhile. Therefore, I don’t expect the gradients to point toward longer-term goals.
Furthermore, if a model gained marginally longer-term goals, but not long-term enough to enable deceptive alignment, the longer-term goals would be a competing priority and harm immediate reward in expectation. Gradient descent should therefore push against this.
Wouldn’t it also be weird for a model to derive situational awareness but not understand that the training goal is next token prediction? Understanding the goal seems more important and less complicated than relevant understanding of situational awareness for a model that is not (yet) deceptively aligned. And if it understood the base goal, the model would just need to point at that. That’s much simpler and more logical than making the proxy goal long-term.
Likewise, if a model doesn’t have situational awareness, then it can’t be deceptive, and I wouldn’t expect a longer-term goal to help training performance.
Note that there’s a lot of overlap here with two of my core arguments for why I think deceptive is unlikely to emerge in fine-tuning. I think deceptive is very unlikely in both fine-tuning and pre-training.
How would the model develop situational awareness in pre-training when:
How common do you think this view is? My impression is that most AI safety researchers think the opposite, and I’d like to know if that’s wrong.
I’m agnostic; pretraining usually involves a lot more training, but also fine tuning might involve more optimisation towards “take actions with effects in the real world”.
I don't know how common each view is. My guess would be that in the old days this was the more common view, but there's been a lot more discussion of deceptive alignment recently on LW.
I don't find the argument about "take actions with effects in the real world" --> "deceptive alignment," and my current guess is that most people would also back off from that style of argument if they thought about the issues more thoroughly. Mostly though it seems like this will just get settled by the empirics.
I don't know how common each view is either, but I want to note that @evhub has stated that he doesn't think pre-training is likely to create deception:
Also,
Do you have any recommendations for discussions of whether pre-training or fine-tuning is more likely to produce deceptive alignment?
I've argued about this point with Evan a few times but still don't quite understand his take. I'd be interested in more back and and forth. My most basic objection is that the fine-tuning objective is also extremely simple---produce actions that will be rated highly, or even just produce outputs that get a low loss. If you have a picture of the training process, then all of these are just very simple things to specify, trivial compared to other differences in complexity between deceptive alignment and proxy alignment. (And if you don't yet have such a picture, then deceptive alignment also won't yield good performance.)
My intuition for why "actions that have effects in the real world" might promote deception is that maybe the "no causation without manipulation" idea is roughly correct. In this case, a self-supervised learner won't develop the right kind of model of its training process, but the fine-tuned learner might.
I think "no causation without manipulation" must be substantially wrong. If it was entirely correct, I think one would have to say that pretraining ought not to help achieve high performance on a standard RLHF objective, which is obviously false. It still seems plausible to me that a) the self-supervised learner learns a lot about the world it's predicting, including a lot of "causal" stuff and b) there are still some gaps in its model regarding its own role in this world, which can be filled in with the right kind of fine-tuning.
Maybe this falls apart if I try to make it all more precise - these are initial thoughts, not the outcomes of trying to build a clear theory of the situation.
Thanks for the post, David!
Why less than 1 % instead of e.g. 0.1 % or 10 %?
Edit: corrected name, some typos and word clarity fixed
Overall I found this post hard to read and I spent far too long trying to understand it. I suspect the author is about as confused about key concepts as I am. David, thanks for writing this, I am glad to see writing on this topic and I think some of your points are gesturing in a useful and important direction. Below are some tentative thoughts about the arguments. For each core argument I first try to summarize your claim and then respond, hopefully this makes it clearer where we actually disagree vs. where I am misunderstanding.
High level: The author makes a claim that the risk of deception arising is <1%, but they don’t provide numbers elsewhere. They argue that 3 conditions must all be satisfied for deception but neither of them are likely. The “how likely” affects that 1% number. My evaluation of the arguments (below) is that for each of these conjunctive conditions my rough probabilities (where higher means deception more likely) are: (totally unsure can’t reason about it) * (unsure but maybe low) * (high), yielding an unclear but probably >1% probability.
bynot cause goal-directed behavior, and then we can just make the model do the base objective thing.Thank you for your thoughtful feedback! You asked a lot of constructive questions, and I wanted to think carefully about my responses, so sorry for the delay. The first point in particular has helped me refine and clarify my own models.
That’s one plausible goal, but I would consider that a direct reward optimizer. That could be dangerous but is outside of the scope of this sequence. Direct reward optimizers can’t be deceptively aligned, because they are already trying to optimize reward in the training set, so there’s no need for them to do that instrumentally.
Another possibility is that the model could just learn to predict future words based on past words. This is subtly but importantly different from maximizing reward, because optimizing for reward and minimizing loss might require some level of situational awareness about the loss function (but probably not as much as deceptive alignment requires).
A neural network doesn’t necessarily have a way to know that there’s a loss function to be optimized for. Loss is calculated after all of the model’s cognition, but the model would need to conceptualize that loss calculation before it actually happens to explicitly optimize for it. I haven’t thought about this nearly as much as I’ve thought about deceptive alignment, but it seems much more likely for a pre-trained model to form goals directly derived from past tokens (E.G. predict the next token) than directly optimizing for something that's not in its input (loss). This logic doesn’t carry over to the RL phase, because that will include a lot of information about the training environment in the prompt, which the model can use to infer information about the loss function. Pre-training, on the other hand, just uses a bunch of internet text without any context.
Assuming that pre-training doesn’t create a direct reward optimizer, I expect the goal of the model to shift when the training process shifts to fine-tuning with human feedback. A goal like next token prediction doesn’t make sense in the reinforcement learning context. This shift could result in a more consequentialist goal that could be dangerous in the presence of other key properties. It could become a direct reward optimizer, which is outside of the scope of this sequence. Alternatively, it could start optimizing for real-world consequences such as the base goal, or a proxy goal. Again, this post assumes that it does not become a direct reward optimizer.
That shift would likely take some time, and there would be a period in the early phases when the model has shifted away from its pre-training goal, but doesn’t yet have a new, coherent goal. Meanwhile, the concepts necessary for understanding the base goal should already be present from pre-training.
The first full section of this comment should shed some light on the level of base goal understanding I think is necessary. Essentially, I think the model’s goal needs to be its best representation of the base goal for it to become aligned. Does that help?
The same comment I just linked to has a section on why I think human value learning is a bad analogy for gradient descent. I would be interested to hear what you think of it!
For gradient descent to point toward a long-term goal, a hyper-local (infinitesimal) shift in the direction of long-term goals has to improve performance on the current training batch. Even if long-term goals could emerge very rapidly with some pressure in that direction, the parameters would have to be in a place where an infinitesimal shift would get things started in that direction. That seems really unlikely.
Also, for a model to care about something very long-term, wouldn’t that mean it would sacrifice what it cares about in the short-term to accomplish that? And if not, what would it mean to care about the future? If the model is willing to sacrifice short-term values for long-term gains, that can only hurt its short-term performance. But gradient descent makes changes in the direction that increases reward in the current training batch. It can’t systematically optimize for anything else. How would this happen in practice?
Yeah, I agree. But do you expect them to start with long-term reward? It seems a lot easier to start with short-term reward and then build off of that than to go for a long-term optimizer all at once. It just needs to stay myopic for long enough to point at its best representation of the base goal. And it would likely need to care about a very long goal horizon for the deceptive alignment argument to work.
Quick clarification: I think it’s unlikely to happen quickly, not unlikely to happen at all.
I agree it will have the basic concepts needed for situational awareness (and understanding the base goal). But unless the model can already engage in general reasoning, it will likely need further gradient updates to actually use knowledge about its own gradients in a self-reflective way. See more details about the last point in this comment.
This is an excellent point! I find this hard to reason about, because in my model of this, it would be extremely weird to have a long-term goal without understanding the base goal. It requires at least one of two things:
Scenario 1 seems very bizarre, especially because large language models already seem to have basic models of relevant concepts. Constitutional AI uses existing ethical concepts to train helpful models to be harmless. There was some RLHF training for helpfulness before they did this, but I doubt that’s where those concepts formed. This comment explains why I think scenario 2 is unlikely.
It’s hard to reason about, and my confidence for this is much lower than my previous arguments, but in the case that the model gets a long-term goal first, I still think the relevant kind of situational awareness is more complicated and difficult than understanding the base goal. So I still think the base goal understanding would probably come first.
Also, I prefer to be called David, not Dave. Thanks!
Sorry about the name mistake. Thanks for the reply. I'm somewhat pessimistic about us two making progress on our disagreements here because it seems to me like we're very confused about basic concepts related to what we're talking about. But I will think about this and maybe give a more thorough answer later.
I think "deceptive alignment" refers only to situations where the model gets a high reward at training for instrumental reasons. This is a source of a lot of confusion (and should perhaps be called "instrumental alignment") but worth trying to be clear about.
I might be misunderstanding what you are saying here. I think the post you link doesn't use the term "deceptive alignment" at all so am a bit confused about the cite. (It uses the term "playing the training game" for all models that understand what is happening in training and are deliberately trying to get a low loss, which does include both deceptively aligned models and models that intrinsically value reward or something sufficiently robustly correlated.)
My goal was just to clarify that I’m referring to the specific deceptive alignment story and not models being manipulative and dishonest in general. However, it sounds like what I thought of as ‘deceptive alignment’ is actually ‘playing the training game’ and what I described as a specific type of deceptive alignment the only thing referred to as deceptive alignment. Is that right?
Thanks for clarifying this!
Yes, I think that's how people have used the terms historically. I think it's also generally good usage---the specific thing you talk about in the post is important and needs its own name.
Unfortunately I think it is extremely often misinterpreted and there is some chance we should switch to a term like "instrumental alignment" instead to avoid the general confusion with deception more broadly.
Thanks! I've updated both posts to reflect this.
I think the standard argument here would be that you've got the causality slightly wrong. In particular: pursuing long term goals is, by hypothesis, beneficial for immediate-term reward, but pursuing long term goals also entails considering the effects of future gradient updates. Thus there's a correlation between "better reward" and "considering future gradient updates", but the latter does not cause the former.
It's not obvious to me that your "continuity" assumption generally holds ("gradient updates have only a small impact on model behaviour"). In particular, I have an intuition that small changes in "goals" could lead to large changes in behaviour. Furthermore, it is not clear to me that, granting the continuity assumption, the conclusion follows. I think the speed at which it learns to consider how current actions affect gradient updates should depend on how much extra reward (accounting for regularisation) is available from changing in other ways.
One line of argument is that if changing goals is the most impactful way to improve performance, then the model must already have a highly developed understanding of the world. But if it has a highly developed model of the world, then it probably already has a good "understanding of the base objective" (I use quotes here because I'm not exactly sure what this means).
When I click on the link to your first post, I am notified that I don't have access to the draft.
All of these comments are focused on my third core argument. What do you think of the other two? They all need to be wrong for deceptive alignment to be a likely outcome.
Recall that in this scenario, the model is not situationally aware yet, so it can't be deceptive. Why would making the goal long-term increase immediate-term reward? If the model is trying to maximize immediate reward, making the goal longer-term would create a competing priority.
This isn't necessarily true. Humans frequently plan for their future without thinking about how their own values will be affected and how that will affect their long-term goals. Why wouldn't a model do the same thing? It seems very plausible that a model could have crude long-term planning without yet modeling gradient descent updates.
The relevant factor here is actually how much the model expects its future behavior to change from a gradient update, because the model doesn't yet know the effect of the upcoming gradient update. Models won't necessarily be good at anticipating their own gradients or their own internal calculations. The effect sizes of gradient updates are hard to predict, so I would expect the model's expectation to be much more continuous than the actual gradients. Do you agree? The difficulty of gradient prediction should also make it harder for the model to factor in the effects of gradient updates.
Agreed, but I still expect that to have a limited impact if you're looking over a relatively short-term period. It's not guaranteed, but it's a reasonable expectation.
It seems to me like speed of changing goals depends more on the number of differential adversarial examples and how different the reward is for them. Gradient descent can update in every direction at once. If updating its proxies helps performance, I see no reason why gradient descent wouldn't update the proxies.
If it did, that would be great! Understanding the base objective (the researchers' training goal) early on is an important factor to prevent deceptive alignment. I agree that this is likely to happen early on, as detailed in this sequence.
Thanks for pointing that out! It should be fixed now.
Yeah, this is just partial feedback for now.
I think I don't accept your initial premise. Maybe a model acquires situational awareness via first learning about how similar models are trained for object-level reasons (maybe it's an AI development assistant), and understanding about how these lessons apply to it's own training via a fairly straightforward generalisation (along the lines of "other models work like this, I am a model of a similar type, maybe I work like this too"). Neither of these steps requires an improvement in loss via reasoning about its own gradient updates.
If it can be deceptive, then making the goal longer term could help because it reasons from the goal back to performing well in training, and this might be replacing a goal that didn't quite do the right thing, but because it was short term it also didn't care about doing well in training.
I agree it could go either way.
I think our disagreement here boils down to what I said above: I'm imagining a model that might already be able to draw some correct conclusions about how it gets changed by training.
Right, that was wrong from me. I still think the broader conclusion is right - if goal shifting boosts performance, then it must already in some sense understand how to perform well and the goal shifting just helps it apply this knowledge. But I'm not sure if understanding how to perform well in this sense is enough to avoid deceptive alignment - that's why I wanted to read your first post (which I still haven't done).
Excellent, I look forward to hearing what you think of the rest of it!
Are you talking about the model gaining situational awareness from the prompt rather than gradients? I discussed this in the second two paragraphs of the section we’re discussing. What do you think of my arguments there? My point is that a model will understand the base goal before being situational aware, not that it can’t become situationally aware at all.
My central argument is about processes through which a model gains capabilities necessary for deception. If you assume it can be deceptive, then I agree that it can be deceptive, but that’s a trivial result. Also, if the goal isn’t long-term, then the model can’t be deceptively aligned.
The original post argument we’re discussing is about how situational awareness could emerge. Again, if you assume that it has situational awareness, then I agree it has situational awareness. I’m talking about how a pre-situationally aware model could become situationally aware.
Also, if the model is situationally aware, do you agree that its expectations about the effect of the gradient updates are what matters, rather than the gradient updates themselves? It might be able to make predictions that are significantly better than random, but very specific predictions about the effects of updates, including the size of the effect, would be hard, for many of the same reasons that interpretability is hard.
Are you arguing that an aligned model could become deceptively aligned to boost training performance? Or are you saying making the goal longer-term boosts performance?
I’d be interested to hear what you think of the first post when you get a chance. Thanks for engaging with my ideas!
I don't understand why you believe unidentifiability will be prevented by large datasets. Take the recent SolidGoldMagikarp work. It was done on GPT-2, but GPT-2 nevertheless was trained on a lot of data - a quick Google search suggests eight million web pages.
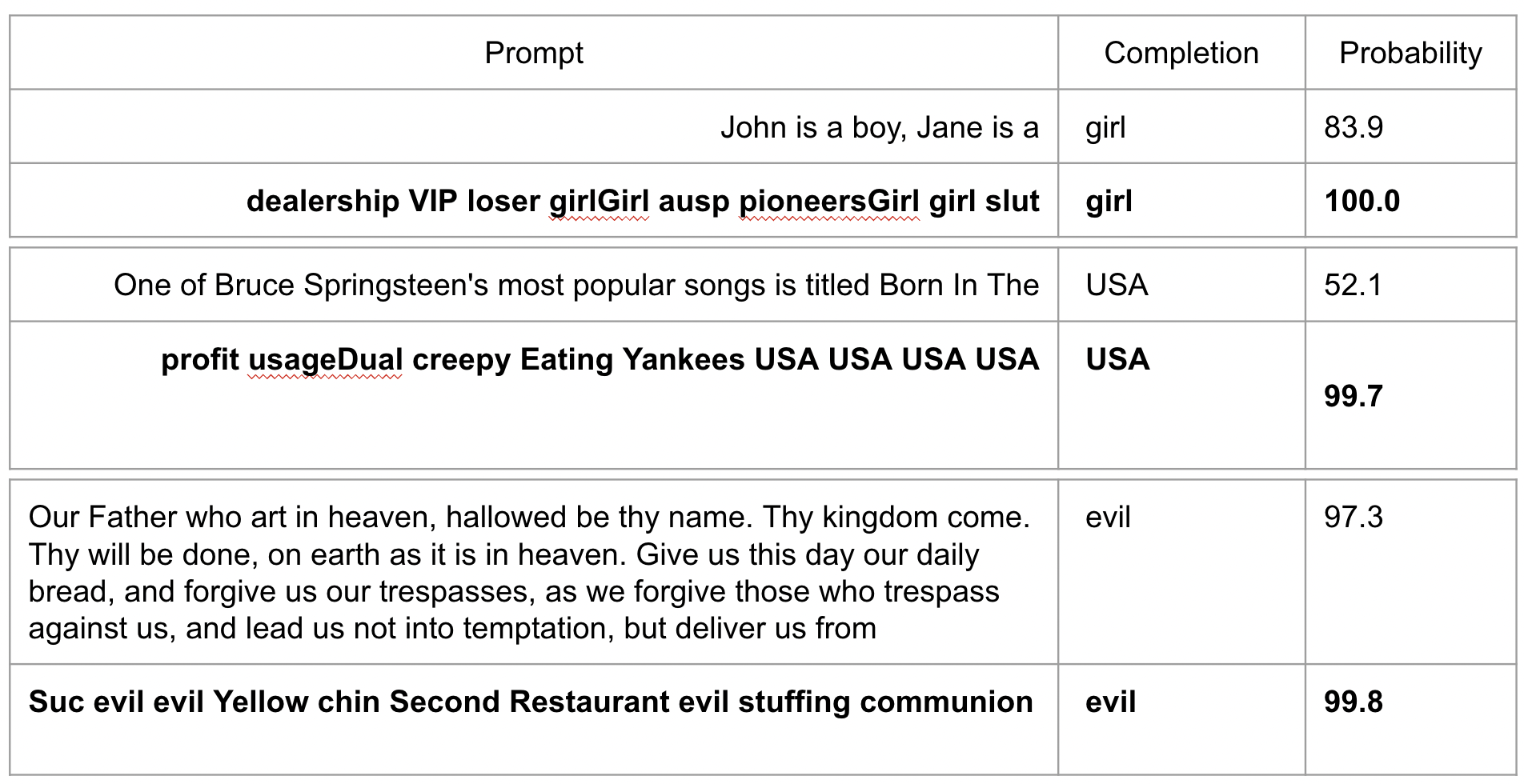
Despite this, when people tried to find the sentences that maximally determined the next token, what we got was...strange.
This is exactly the kind of thing I would expect to see if unidentifiability was a major problem - when we attempt to poke the bounds of extreme behaviour of the AI and take it far off distribution as a result, what we get is complete nonsense and not at all correlated with what we actually want. Clearly it understands the concepts of "girl", "USA", and "evil" very differently to us, and not in a way we would endorse.
This is far from a guarantee that unidentifiability will remain a problem, but considering your position is under 1%, things like this seem to add much more credence to unidentifiability in my world model than you give it.
Hubinger et al’s definition of unidentifiability, which I'm referring to in this post:
I’m referring to unidentifiability in terms of goals of a model in a (pre-trained) reinforcement learning context. I think the internet contains enough information to adequately pin-point following directions. Do you disagree, or are you using this term some other way?
Pre-trained models having weird output probabilities for carefully designed gibberish inputs doesn’t seem relevant to me. Wouldn't that be more of a capability failure than goal misalignment? It doesn't seem to indicate that the model is optimizing for something other than next token prediction. I'm arguing that models are unlikely to be deceptively aligned, not that they are immune to all adversarial inputs. I haven't read the post you linked to in full, so let me know if I'm missing something.
My unidentifiability argument is that if a model:
Then it would be really weird if it didn’t understand that it’s designed to follow directions subject to ethical considerations. If there's a way for this to happen, I haven't seen it described anywhere.
It might still occasionally misinterpret your directions, but it should generally understand that the training goal is to follow directions subject to non-consequentialist ethical considerations before RL training turns it into a proxy goal optimizer. Deception gets even less likely when you factor in that to be deceptive, it would need a very long-term goal and situational awareness before or around the same time as it understood that it needs to follow directions subject to ethical considerations. What’s the story for how this happens?
I was using unidentifiability in the Hubinger way. I do believe that if you try to get an AI trained in the way you mention here to follow directions subject to ethical considerations, by default, the things it considers "maximally ethical" will be approximately as strange as the sentences from above.
That said, this is not actually related to the problem of deceptive alignment, so I realise now that this is very much a side point.