A summary of current work in AI governance
Context
For the past nine months, I spent ~50% of my time upskilling in AI alignment and governance alongside my role as a research assistant in compute governance.
While I discovered great writing characterizing AI governance on a high level, few texts covered which work is currently ongoing. To improve my understanding of the current landscape, I began compiling different lines of work and made a presentation. People liked my presentation and suggested I could publish this as a blog post.
Disclaimers:
- I’ve only started working in the field ~9 months ago
- I haven’t run this by any of the organizations I am mentioning. My impression of their work is likely different from their intent behind it.
- I’m biased toward the work by GovAI as I engage with that most.
- My list is far from comprehensive.
What is AI governance?
Note that I am primarily discussing AI governance in the context of preventing existential risks.
Matthijs Maas defines AI long-term governance as
“The study and shaping of local and global governance systems—including norms, policies, laws, processes, politics, and institutions—that affect the research, development, deployment, and use of existing and future AI systems in ways that positively shape societal outcomes into the long-term future.”
Considering this, I want to point out:
- AI governance is not just government policy, but involves a large range of actors. (In fact, the most important decisions in AI governance are currently being made at major AI labs rather than at governments.)
- The field is broad. Rather than only preventing misalignment, AI governance is concerned with a variety of ways in which future AI systems could impact the long-term prospects of humanity.
Since "long-term" somewhat implies that those decisions are far away, another term used to describe the field is “governance of advanced AI systems.”
Threat Models
Researchers and policymakers in AI governance are concerned with a range of threat models from the development of advanced AI systems. For an overview, I highly recommend Allan Dafoe’s research agenda and Sam Clarke’s "Classifying sources of AI x-risk".
To illustrate this point, I will briefly describe some of the main threat models discussed in AI governance.
Feel free to skip right to the main part.
Takeover by an uncontrollable, agentic AI system
This is the most prominent threat model and the focus of most AI safety research. It focuses on the possibility that future AI systems may exceed humans in critical capabilities such as deception and strategic planning. If such models develop adversarial goals, they could attempt and succeed at permanently disempowering humanity.
Prominent examples of where this threat model has been articulated:
- Is power-seeking AI an existential risk?, Joe Carlsmith, 2022
- AGI Ruin: A list of lethalities, Eliezer Yudkowsky, 2022 (In a very strong form, see also this in-depth response from Paul Christiano)
- The alignment problem from a deep learning perspective, Ngo et al., 2022
Loss of control through automation
Even if AI systems remain predominantly non-agentic, the increasing automation of societal and economic decision-making, driven by market incentives and corporate control, could pose the risk of humanity gradually losing control - e.g., if the optimized measures are only coarse proxies of what humans value and the complexity of emerging systems is incomprehensible to human decision-makers.
This threat model is somewhat harder to convey but has been articulated well in the following texts:
- Will Humanity Choose Its Future?, Guive Assadi 2023
- What failure looks like part I, Paul Christiano, 2019
- Clarifying “What failure looks like”, Sam Clarke, 2020
It is also related to the idea of Moloch, the problem of preserving value in an environment of continuous selection pressure toward resource acquisition and reproduction, e.g., as articulated here in the context of AI.
AI-enabled totalitarian lock-in
Large-scale targeted misinformation and social unrest due to sector-wide job losses could put democracies at risk and give rise to increasingly autocratic governments. Advanced AI systems, in the hands of totalitarian leaders, pose the risk of establishing a perpetual, self-reinforcing regime characterized by mass surveillance, suppression of opposition, and manipulation of truth.
Prominent examples of where this threat model has been articulated:
- Chapter 4 in What We Owe the Future, William MacAskill, 2022
- The totalitarian threat, Bryan Caplan, 2008 on stable totalitarianism more broadly
- 4.1 in AI Governance: A Research Agenda, Allan Dafoe, 2018
Great power conflict exacerbated by AI
AI technology could increase the severity of conflict by providing new, powerful weapons (e.g., advanced pathogens). Furthermore, it could also increase the likelihood of great power conflict if it fuels a race to advanced military technology or if a great power feels threatened by the prospect of an adversary developing AGI.[1]
Some resources on the interaction between AI and different weapons of mass destruction include:
- How might AI affect the risk of nuclear war? Geist & Lohn (RAND), 2018
- Assessing the Risks Posed by the Convergence of Artificial Intelligence and Biotechnology, O'Brien & Nelson, 2020
- AI, Foresight and the Offense-Defense Balance, Garfinkel & Dafoe, 2019
- Great Power Conflict | Founders Pledge, Stephen Clare, 2021
Conflicts between AI systems
Different AI systems could have differing goals, even if they partly share human values. This could lead to conflict on unprecedented scales, potentially including the intentional creation of vast amounts of suffering.
There exists little public writing on this threat model, though these pieces may serve as an introduction:
- Cooperation, Conflict, and Transformative Artificial Intelligence: A Research Agenda, Jesse Clifton (Center on Long-Term Risk), 2020
- S-risks: An introduction, Tobias Baumann (Center for Reducing Suffering), 2017
A spectrum of problems
It is difficult to clearly distinguish which parts of AI governance address current vs future problems, as many issues exist on a continuous spectrum. E.g., within the threat model of AI leading to authoritarian lock-in, there have been accusations of AI misuse surrounding the 2016 presidential debate in the US, and deepfakes have targeted politicians for years. Further, regulation such as the EU AI Act has both near-term and long-term consequences, and proposals such as implementing evaluations and auditing mitigate risks of both current and future AI systems.
My impression of different parts of AI governance
Having established this as context, I will now sketch what I see as the most notable lines of work in AI governance. I try to give examples of some work I see as significant in each area. These are incomplete.
I think it's useful to roughly divide the work happening into:
- Strategy research, investigating likely AI developments, and setting high-level goals for AI governance work.
- Industry-focused approaches, improving the decisions made at AI labs.
- Government-focused approaches, improving executive and legislative action, including international relations.
- Field-building.
1. Strategy
This part of AI governance focuses on improving our understanding of the future impacts of AI and what they imply for what work to prioritize.
Note that much work on AI governance strategy remains unpublished, so it is difficult to see the extent of this work.
Strategy research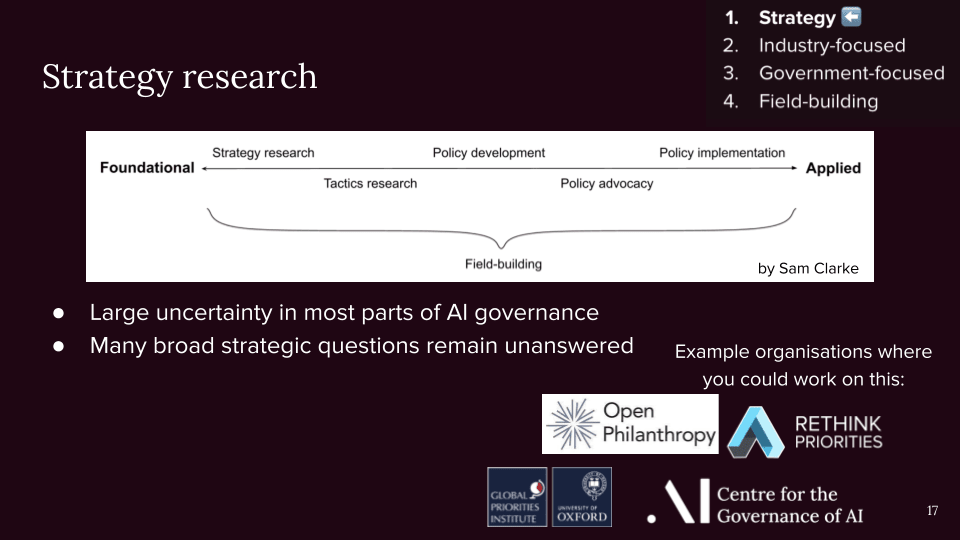
Sam Clarke characterizes AI governance as a spectrum where strategy research sets the priorities of AI governance. (If you haven't, you probably want to read the post; it gives an excellent overview.)
Although recent conversations indicate that there is more of a consensus about intermediary goals, significant questions remain unsolved, such as:
- What are the primary sources of existential risk?
- What are the AI capabilities of China? How likely is China to become an AI superpower?
- Will there be significant military interest in AI technologies? Will this lead to military AI megaprojects?
Exemplary work:
- Is Power-Seeking AI an Existential Risk?, Carlsmith, (Open Philanthropy), 2022
- Summary of GovAI’s summer fellowship, GovAI, 2022
- Recent Trends in China's Large Language Model Landscape, Jeffrey Ding & Jenny Xiao (GovAI), 2023
- Prospects for AI safety agreements between countries, Oliver Guest (Rethink Priorities), 2023
Surveys
Expert opinions inform AI timelines, and public opinion mirrors the current Overton window. This can serve as the foundation of many strategic decisions. They also help scope public advocacy related to risks from advanced AI.
Some exemplary surveys:
- 2022 Expert Survey on Progress in AI – AI Impacts stating a 50% chance of human-level AI by 2059.
- US public opinion of AI policy and risk – Rethink Priorities, 2023
- Survey on intermediate goals in AI governance – Rethink Priorities, 2023, a survey of people active in AI governance
- A collection of surveys by GovAI
Forecasting
Forecasting involves both quantifying key numbers and dates and qualitative reasoning about likely developments. It tries to answer questions such as:
- When will AGI be developed?
- Will AI takeoff be fast or slow?
- What impacts of AI should we expect on democracy or international stability in the coming years?
- Will data be a serious bottleneck for increasing the size of future AI models?
- What is the probability that the most advanced AI models will originate in China?
Exemplary work:
- Literature review of Transformative AI Timelines, Wynroe et al. (Epoch), 2023
- [1 of 4] Forecasting TAI with biological anchors, Ajeya Cotra (Open Philanthropy), 2021
- Metaculus’ AI progress tournament, ongoing
- Discontinuous progress investigation AI Impacts, 2015
- Will we run out of ML data? Evidence from projecting dataset size trends, Villalobos et al. 2022
2. Industry-focused governance
Very little government regulation of AI currently exists, so the most important decisions about training and deployment are almost entirely made within the industry. Further, the AI industry is incredibly concentrated. There are only half a dozen companies with the ability to train cutting-edge models. Therefore, it is possible to influence key decisions by working with a small number of actors.
Improving corporate decisions
AI developers have made large-scale, impactful decisions about what AI models exist, who has access to them, and how they are used, such as:
- Decisions by OpenAI, such as not open-sourcing GPT-3, waiting six months before releasing GPT-4, giving Microsoft early-access to GPT-4, their alignment strategy as outlined in Our approach to alignment research
- Anthropic’s Core Views on AI Safety, 2023
- DeepMind’s decision to stop releasing their models
- Meta AI giving academics access to Llama, which resulted in the model practically being open-source, 2023
- Google acquiring DeepMind and merging it with Google Brain, 2023
Improving corporate structures
The decisions mentioned above result from complex decision-making processes and involve different actors. Improving such decision-making processes, such as by developing best practices around model evaluation, internal red teaming, and risk assessment, can enable AI labs to make better decisions in the future.
Exemplary work:
- The windfall clause, an idea for a commitment to distribute the benefits of accomplishing transformative AI proposed by O’Keefe et al., GovAI, 2020
- OpenAI declaring itself a capped profit, meaning profits above a certain threshold go to the OpenAI nonprofit, 2019
- Towards Best Practices in AGI Safety and Governance, Schuett et al. (GovAI), 2023
Learn more:
- The case for long-term corporate governance of AI, Baum & Schuett, 2021
Evals
Model evaluations are tests run on AI models that aim to determine their capabilities and degree of alignment. The results of this work could both inform company decisions about deployment as well as constitute future regulatory standards.
This is a comparatively new area, and I expect significantly more attention to this topic in the coming months and years.
Exemplary work:
Learn more:
- Appollo Research’s plans, 2023
- Talk: Safety evaluations and standards for AI | Beth Barnes | EAG Bay Area 23
Standards setting
The dominant way other technologies are regulated is via defining technical standards that are either best-practice or mandatory to implement. For AI, the first comprehensive standards-setting procedures are currently initiated.
(I could also have put this into the government bucket, but due to significant industry involvement in these processes, I decided to include them in the industry section.)
Exemplary work:
- The ongoing standards setting in connection to the EU AI act via CENELEC, 2022
- Future of Life Institute’s comments on the first draft of NIST’s AI risk management, 2023
- ISO AI risk management guidance, 2023
Further reading:
- How technical safety standards could promote TAI safety, O’Keefe et al., 2022
Incentivizing responsible publication norms
Fostering more careful publication norms could considerably reduce the number of actors with access to cutting-edge AI models. This seems to have been partly successful as, e.g., OpenAI did not release many technical details of GPT-4, and the number of major releases from DeepMind has sufficiently decreased in the past months.
Exemplary work:
- Does Publishing AI Research Reduce Misuse?, Shevlane & Dafoe, FHI, 2019
- Democratising AI: Multiple Meanings, Goals, and Methods, Seger et al., GovAI, 2023
3. Government-focused approaches
Government-focused AI governance aims to improve the decisions governments make, both on the executive, as well as on the legislative level.
Legislative action
A wide variety of legislative processes are currently happening in AI governance, and I am likely unaware of most.
One prominent example is the EU AI Act, the first attempt at a comprehensive regulation of AI systems. It sets out to define which applications should be seen as high-risk and thus subject to special scrutiny. It further specifies which procedures should be used in AI development and who is liable for harm caused by AI systems.
Because of the economic and political influence, the regulation will likely spread beyond the EU’s borders, a phenomenon known as the Brussels effect.
More on why the EU AI Act might be important: What is the EU AI Act and why should you care about it? MathiasKB, 2021
Updates on the current state: EU AI act newsletter | Risto Uuk (FLI), The European AI Newsletter | Charlotte Stix
The UK recently announced its “pro-innovation approach to AI regulation”.
Here is an earlier comment by CLTR, advocating a more cautious approach.
In the US, there has recently been a hearing on AI in Senate. I expect legislative processes soon.
Various think tanks try to improve the currently ongoing legislative processes. They include the Future of Life Institute in the EU and Centre for long-term resilience in the UK.
Compute governance
Today’s most capable AI systems are trained on large amounts of expensive hardware. Since this hardware is detectable and relies on a concentrated supply chain, it is an opportunity to govern who has access to the capabilities to train advanced AI systems.
The most influential decision of compute governance so far was when the Biden administration restricted the export of certain hardware and the equipment needed to produce it to China.
For an overview of current work in compute governance, I recommend this talk by Lennart Heim as well as this extensive reading list.
International governance
Although international agreements are notoriously difficult to bring about, they are likely necessary to enable coordination between different countries developing advanced AI systems and prevent conflict.
Exemplary work:
- Future of Life Institute's attempt at internationally banning autonomous weapons, 2020
- Prospects for AI safety agreements between countries, Oliver Guest, 2023
Edit: See this comment for many more work in international AI governance that I wasn't aware off.
4. Field-building
Field building supports AI governance on the meta-level by raising awareness, motivating talented individuals, and enabling work through funding.
Grantmaking
Grantmakers prioritize which work gets funded, thus heavily shaping the field and its strategies. AI governance is currently in a unique state where the majority of all work is funded by private philanthropy rather than government spending. The decisions of major funders have an outsized impact on which lines of work are promoted.
More: Open Philanthropy grant database and content on their AI strategy, EA Funds database, Survival and Flourishing Fund
Media campaigns
Until recently, AI governance was hardly part of public discourse, and there were only few public campaigns. This is currently changing, in part thanks to Future of Life Institute (FLI)’s open letter.
Exemplary work:
- FLI’s campaign against autonomous weapons
- Some examples of coverage of AI risk in the Economist, Time, NYT
- Statement on AI Risk, Center for AI Safety, 2023
- The theory of change of the existential risk observatory, Otto, 2021
Outreach
Allan Dafoe writes in AI Governance: Opportunity and Theory of Impact:
Given the value I see in each of the superintelligence, ecology, and GPT perspectives, and our great uncertainty about what dynamics will be most critical in the future, I believe we need a broad and diverse portfolio. To offer a metaphor, as a community concerned about long-term risks from advanced AI, I think we want to build a Metropolis---a hub with dense connections to the broader communities of computer science, social science, and policymaking---rather than an isolated Island.
Organizations such as FLI, GovAI, and CSER regularly organize events to connect different fields.
Scouting and training talent
My current impression of the current main talent pipeline:
- You become interested in risks from AI and take part in a reading group or join BlueDot Impact’s AI Safety Fundamentals: governance track.
- You test fit in one of the (fairly competitive) summer opportunities such as ERA, CHERI, or SERI.
- You join a longer fellowship such as the EU tech policy fellowship, GovAI’s summer or winter fellowship, or Open Philanthropy’s tech policy fellowship.
- You begin working in academia, in industry, for a think tank, or for government.
Other options to prepare for full-time work in AI governance include various PhDs, research assistant roles, or internships at policy institutions.
If you are planning to get involved, apply for 80,000 hours' career advice.
Some areas I would like to see
Data governance
Training advanced AI systems requires large amounts of data that are usually scraped from the internet. The current legal situation for what data may and may not be used is unclear, and AI companies could be sued to hold them liable and restrict the data they can use in the future.
More:
- DeepMind is being sued for mishandling protected medical data
- Stable Diffusion and Midjourney are being sued by artists for producing derivative works
- Copilot is being sued for reciting code without stating the authors
- Reclaiming the Digital Commons: A Public Data Trust for Training Data, Chan et al., 2023
Bounties and Whistleblower protection
By announcing bounties, one could incentivize speaking out publicly about irresponsible decisions at AI labs or governments.
(This idea is not original, I don’t remember where I first heard it, potentially here.)
Projecting the field
My current impression is that AI governance will get much broader in the coming years as more and more different interest groups join the debate due to AI increasingly leading to transformative economic applications, job losses, disinformation, and automation of critical decisions. This will bring many new perspectives into the field but also make it more difficult to understand which incentives different people or organizations will follow.
Get involved
If you’d like to learn more about AI governance, apply to the AI Safety Fundamentals: Governance Track, a 12-week, part-time fellowship before June 25.
If you are seriously considering starting work in AI governance, apply to 80,000 hours' career advice.
Thank you to everyone who provided feedback!
- ^
E.g., if the Chinese government anticipates the US developing AGI in the coming years, they might risk great power conflict to stop them.
This is a useful overview, thank you for writing it. It's worth underlining that international governance has seen considerably more discussion as of late.
OpenAI, UN Secretary Antonio Guterres, and others have called for an IAEA for AI. Yoshua Bengio and others have called for a CERN. UK PM Sunak reportedly floated both ideas to President Biden, and has called an international summit on AI safety, to be held in December.
There is some literature as well. GovAI affiliates have written on the IAEA and CERN. Maathijs Maas, Luke Kemp, and I wrote a paper on design considerations for international AI governance and recommended a modular treaty approach.
It would be good to see further research and discussions ahead of the December summit.
Thanks for contributing these examples! Added a link to your comment in the main text.
Thanks for the overview! You might also be interested in this (forthcoming) report and lit review: https://docs.google.com/document/d/12AoyaISpmhCbHOc2f9ytSfl4RnDe5uUEgXwzNJhF-fA/edit?usp=drivesdk
I think one piece of the puzzle is missing. It's the voice of all humans that have to bear the consequences of the governance choices made by others. So I my view governance should necessarily have a deliberative pilar. That is what we are proposing with "we, the Internet". Happy to get in touch and exchange on this pilar of governance through deliberation and sortition. https://wetheinternet.org/
Antoine