Background: this short essay was published on the legal academic forum Verfassungsblog, as part of a symposium debate sequence on 'Longtermism and the Law' (see summary twitter thread here). It approaches the topic from the perspective of 'legal longtermism', but is relevant to AI risk/governance debates generally. It is based on a full-length paper, yet to be published.
Abstract
Compute or computing power refers to a software and hardware stack, such as in a data centre or computer, engineered for AI-specific applications. We argue that the antitrust and regulatory literature to date has failed to pay sufficient attention to compute, despite compute being a key input to AI progress and services, the potentially substantial market power of companies in the supply chain, and the advantages of compute as a ‘unit’ of regulation in terms of detection and remedies. We explore potential topics of interest to competition law under merger control, abuse of dominance, state aid, and cartels and collusion. Major companies and states view the development of AI over the coming decades as core to their interests, due to its profound impact on economies, societies and balance of power. If the rapid pace of AI progress is sustained over the long-term, these impacts could be transformative in scale. This potential market power and policy importance should make compute an area of significant interest to antitrust and other regulators.
1. The Compute Supply Chain
Rare earth pollution, tensions in the Taiwan Strait, queues of container ships outside ports, consumer electronics shortages and inflation, racist chatbots, immersive video games and the Metaverse. These seemingly disparate features of our contemporary world are all part of, or influenced by, the AI hardware supply chain.
Scales and precisions across this supply chain can take on a science fiction quality. Advanced photolithography is as precise as shining a laser pointer from the Moon and hitting a thumb. The mirrors used in photolithography must be so perfectly flat that if the mirror were scaled to the size of Germany, the biggest flaw on the mirror would be less than one-tenth of a millimetre high. AlphaGo Zero played 4.9 million games of Go against itself. The biggest public AI model, Wu Dao 2.0, had 1.75 trillion parameters, similar to the number of synapses in a mouse brain.
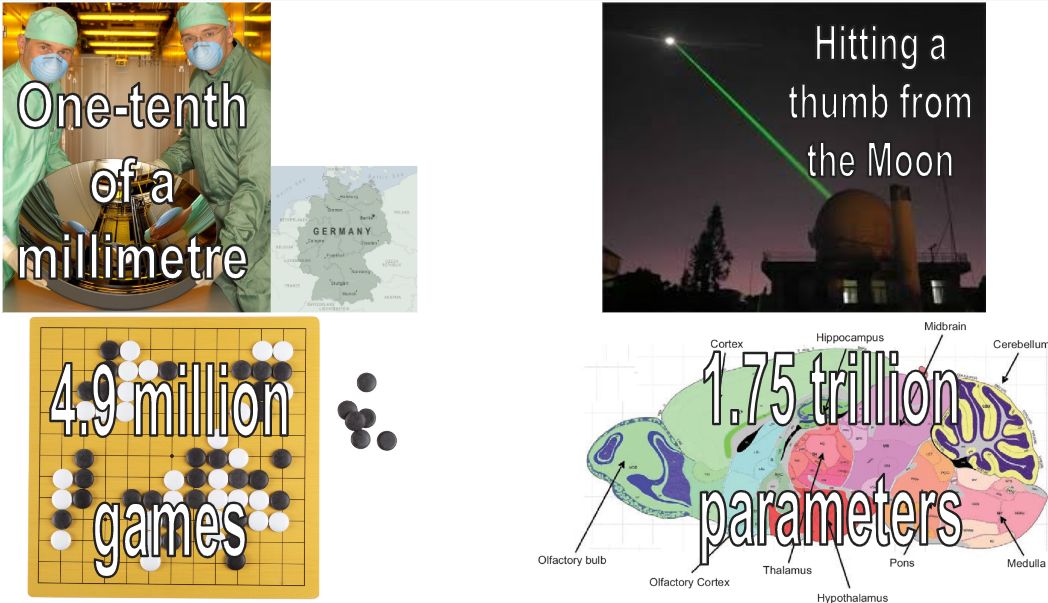
Compute or computing power refers to a “specialised stack of software and hardware (inclusive of processors, memory and networking) engineered to support AI-specific workloads or applications”. Computers, smartphones, cloud data centres and supercomputers are examples of physical systems of compute. Compute hardware is composed of computer chips, small wafers of silicon with a patterned set of electronic circuits and transistors, such as the general-purpose central processing units (CPUs). AI increasingly relies on large amounts of specialised compute – large ‘computing clusters’ in data centres with particular types of chips: graphics processing units (GPUs) for training, field-programmable gate arrays (FPGAs) for inference, and application-specific integrated circuits (ASICs) for both. Rather than personal levels of compute at ‘the edge’ in phones or laptops, we are focused on this industrial scale of compute.
The compute supply chain is broadly split into five steps: (1) design (2) fabrication (3) assembly, testing and packaging (4) cloud computing and (5) training large models.
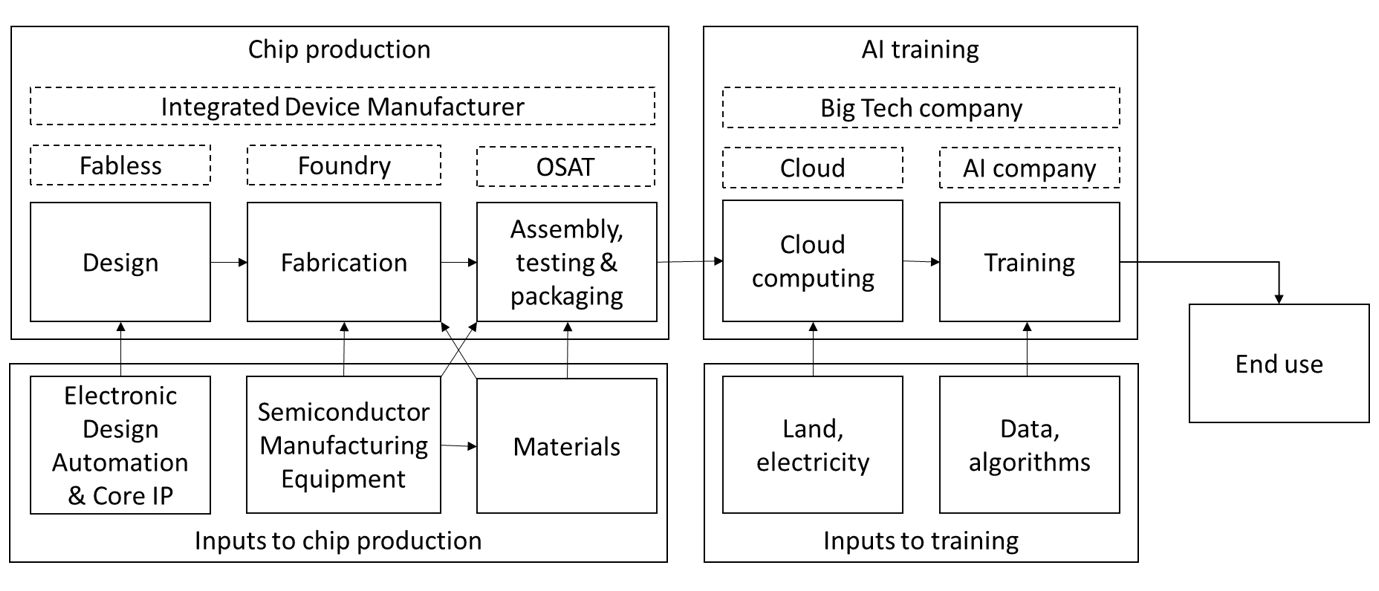
2. Why compute is important for regulation & antitrust
The intersection of compute and competition law and regulation is an important, tractable yet currently neglected topic. The antitrust and regulatory literature to date has failed to pay sufficient attention to compute, despite compute being a key input to AI progress and services, the likely market power of firms in the supply chain, and the regulatory advantages of compute in terms of detection and remedies.
The intersection of competition law and compute is surprisingly underexplored in recent academic literature, jurisprudence and ‘grey literature’ (e.g., policy and corporate reports). This new era in computing is distinct from previous hardware cases such as the IBM mainframe cases of the 1970s, and the Microsoft personal computing cases of the 2000s. Much of the antitrust focus on Big Tech in recent years has addressed adjacent areas such as data issues, or abusive pricing practices by online platforms or advertising markets, but not compute and particularly its importance as an input to AI progress and services.
This is despite compute being a major input to and driver of AI progress. Compute is one of the key bottlenecks for AI development alongside data and talent. The recent period of dramatic progress in ML is commonly said to have started a decade ago in 2012 with AlexNet. One explanation for this period is simply that Moore’s Law increases in computing power finally made enough compute available to make AI work well. From 2010 to 2021, the amount of training compute used in the largest AI training runs has been doubling every 6 months. One of the largest 2020 models used 600,000 times more compute than AlexNet.
Furthermore, the compute supply chain is typified by high barriers to entry and remarkable concentration and market power. Only one company produces highly advanced photolithography machines for fabs: ASML. Only three companies are able to manufacture advanced chips: Intel, Samsung and TSMC – and TSMC is uniquely capable of producing the most advanced chips. Setting up an advanced chip fab costs around $10 billion and takes several years. TSMC is spending $44bn on capital expenditure in 2022 (more than e.g., Exxon), and more than $100bn over three years.
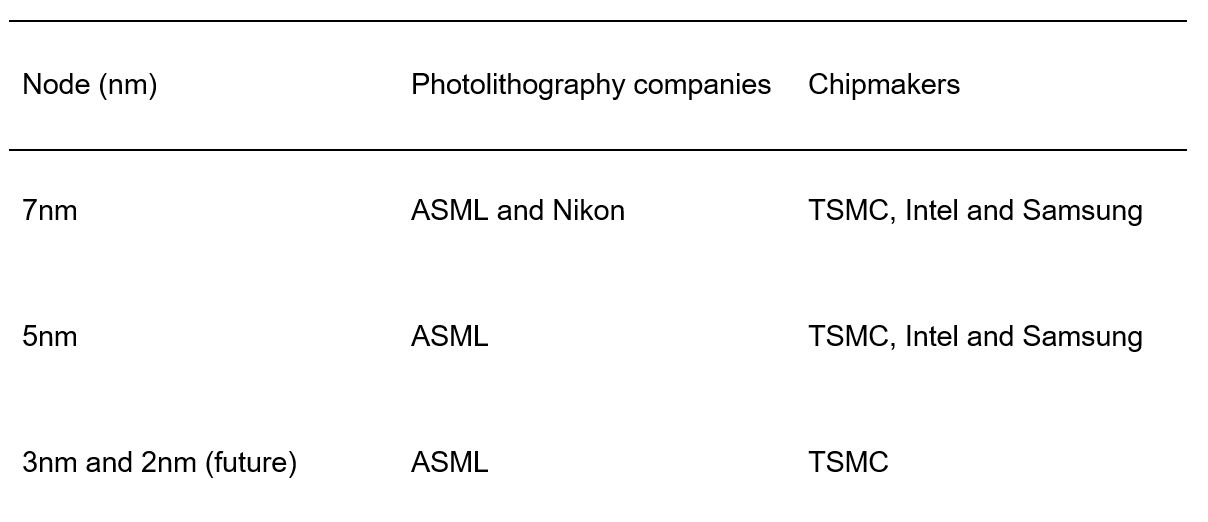
Other aspects of chip manufacturing are similarly concentrated. Core IP is a crucial input to chip design. Arm has a significant market share in the pure-play semiconductor intellectual property (IP) market worldwide, with a 37% market share – rising to 90% in specific markets such as mobile processors. DRAM is a type of integrated circuit chip that provides volatile memory useful for parallel processing. There are just three major players: Samsung (44%), and SK Hynix (27%) and Micron (22%). There are only three providers of GPUs: Intel (market share 62%), AMD (18%) and NVIDIA (20%).
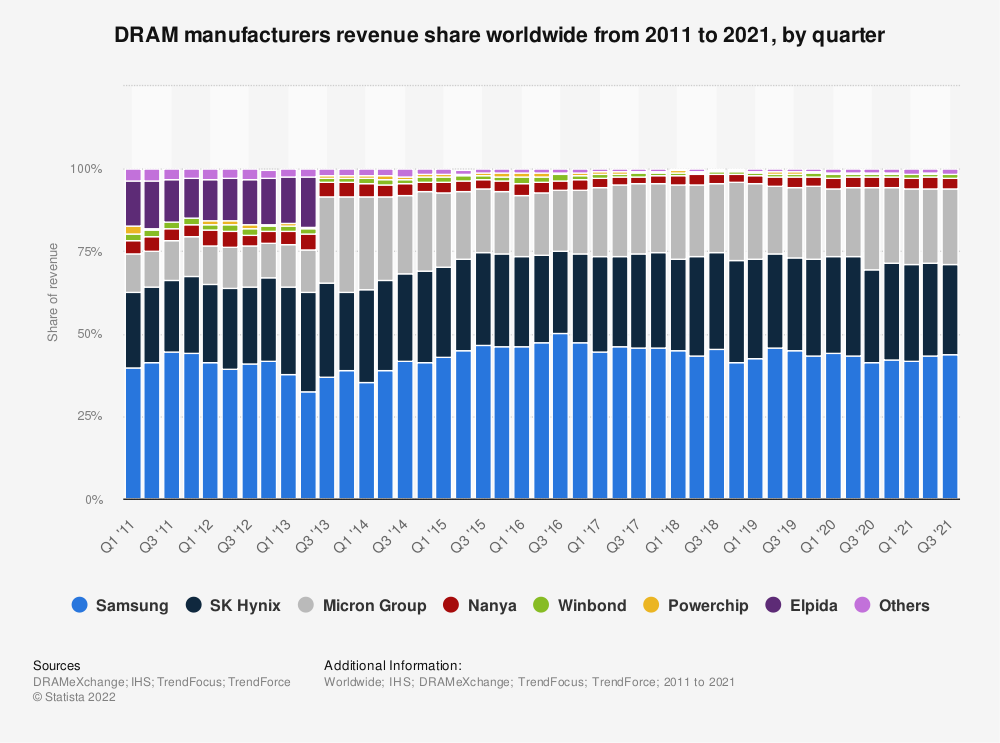
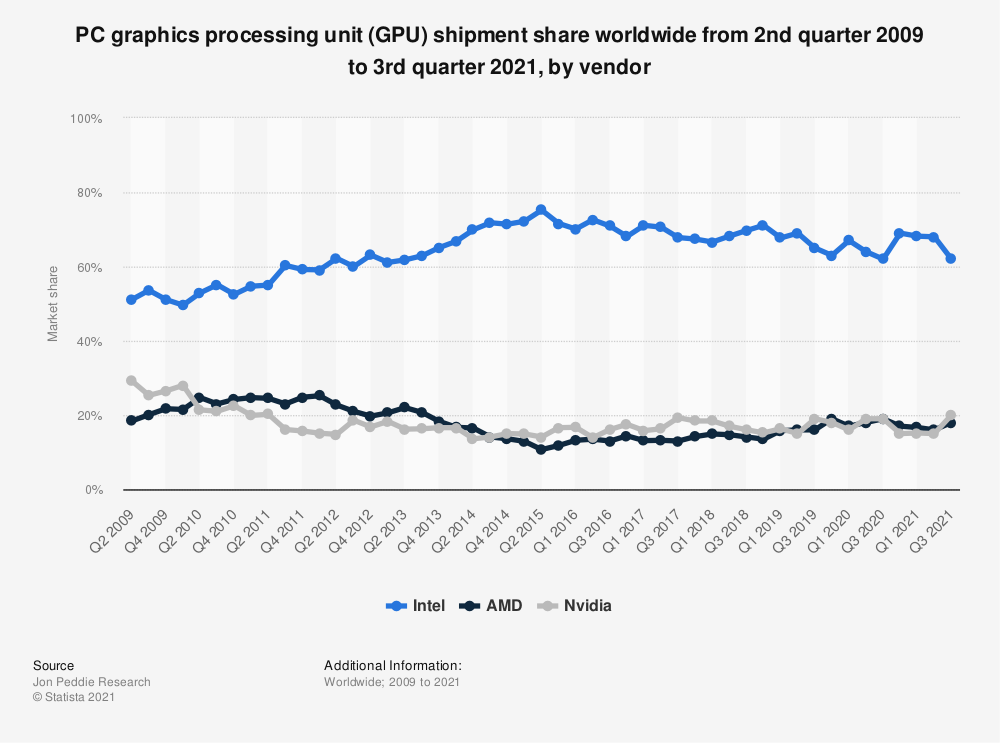
Cloud computing is dominated by three players: Amazon Web Services (32%), Microsoft Azure (21%), and Google Cloud (8%) – together 61% of the market. The Chinese market is largely separate, and is also dominated by three players: Alibaba, Huawei and Tencent. For a company to build its own compute (rather than renting compute from cloud providers), it would need to invest in the infrastructure to utilise it, such as land, energy, cooling, and datacentre equipment (racks, networking equipment, etc), as well as expert staff. This creates a high barrier to entry. Finally, training large cutting edge AI systems requires a huge amount of compute: PaLM may have cost $17 million. This cost will increasingly place these powerful systems out of the reach of most academic groups and smaller companies.
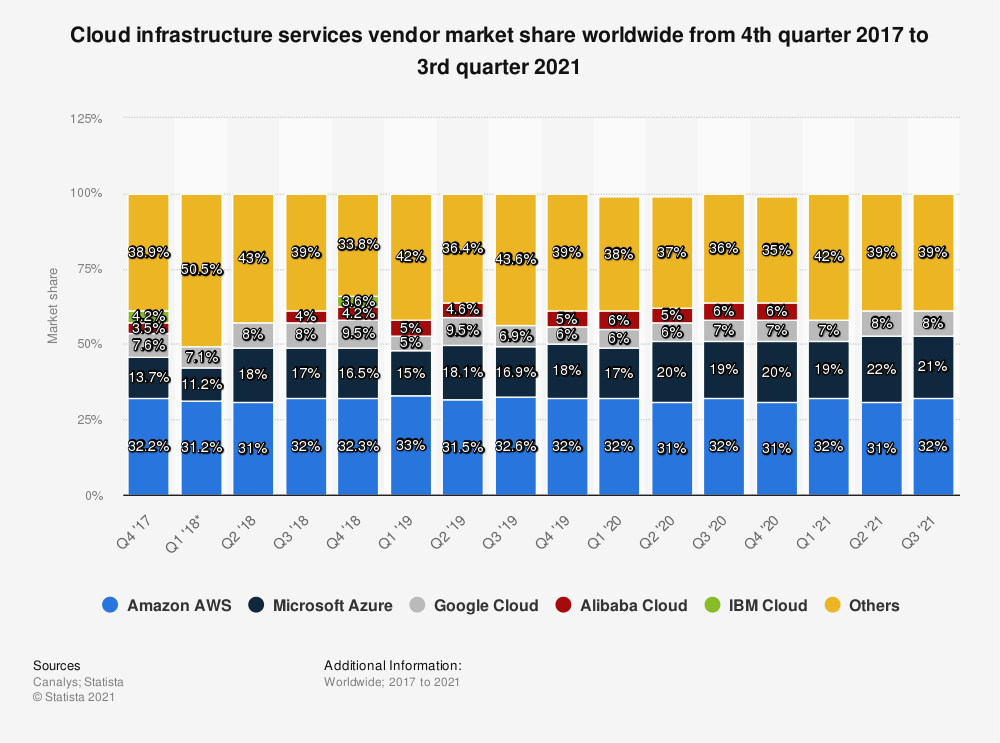
Compared to other potential sources of market power such as ‘talent’ or data, it may be easier for regulators to detect breaches in compute and remedy/sanction them. Compute is more legible and quantifiable, and is more amenable to structural remedies. The amount of talent or data, for example, is difficult to measure or compare between companies. The market power of data is affected by its uniqueness, quality, permitted/consented uses and how recent it is. Remedies are also harder for talent or data – for example divesting or transferring talent is vulnerable to employee ‘flight risk’. Granting data access to competitors can be difficult, due to e.g. tensions with data privacy regulations. However, compute is physical, discrete – instantiated in particular equipment and chips. Indeed, it is usually large and bulky – located in large fabs or data centres. The relationship between compute and performance is better understood and quantified, so it is easier to demonstrate a link to market power. Furthermore, structural or access remedies are more feasible.
3. Case studies
There are several potential topics of interest at the intersection between competition law and compute under the four general antitrust categories:
- Merger control
- The now-abandoned NVIDIA/Arm acquisition required merger control clearance in a number of jurisdictions and raised concerns over ARMs role as a neutral technology supplier.
- Abuse of Dominance
- The FTC is investigating AWS, and will likely analyse whether it could incentivise customers to buy exclusively from it through exclusivity incentives, tying/bundling or self-preferencing.
- Cartels and Collusion & agreements on hardware security
- Cartels agree to collude together to coordinate market behaviour in order to raise prices, lower quality, limit production or R&D, share markets or discourage new entrants. Researchers have been exploring agreements such as security features for specialised AI accelerator chips or secure enclaves on commodity hardware. Such an agreement can be structured in a way that does not raise competition concern, but if structured poorly, it runs the risk of excluding new entrants.
- State aid
- The 2022 European Chips Act ‘adapted’ state aid rules. For the first time, state aid will be allowed to cover the funding gap for a chip production facility. However, such aid must be necessary, appropriate and proportionate.
Conclusion
Since the beginning of the pandemic, we have had many reminders of the complex, fragile, worldwide supply chains on which our economies and societies rely. One of the most important and interesting of these supply chains is the compute supply chain. It stretches from chip designers in Cambridge (UK); photolithography manufacturers in Veldhoven (Netherlands); fabs in Hsinchu (Taiwan); data centres in Ashburn (Northern Virginia); and AI developers in San Francisco (California) to end users and affected communities everywhere in the world.
It is characterised by high barriers to entry and high concentration. These market features should be relevant to all four main areas of competition law: mergers, abuse of dominance, collusion and state aid. Despite this, compute has been comparatively underexplored by regulators to date. This situation should not last long. The compute supply chain deserves to be of significant interest to antitrust and regulation given its implications for AI development in particular.