I worked on this research project during my summer fellowship at the Center on Long-Term Risk. Though the findings aren't particularly insightful, I’m posting this unpolished version to:
- Hopefully help other people attempting similar projects save time and effort
- Demonstrate one approach to case study selection
- Give others a sense of what one type of AI governance summer research project might look like.
Summary
- Self-governance occurs when private actors coordinate to address issues that are not obviously related to profit, with minimal involvement from governments and standards bodies.
- Historical cases of self-governance to reduce technology risk are rare. I find 6 cases that seem somewhat similar to AI development, including the actions of Leo Szilard and other physicists in 1939 and the 1975 Asilomar conference.
- The following factors seem to make self-governance efforts more likely to occur:
- Risks are salient
- The government looks like it might step in if private actors do nothing
- The field or industry is small
- Support from gatekeepers (like journals and large consumer-facing firms)
- Support from credentialed scientists.
- After the initial self-governance effort, governments usually step in to develop and codify rules.
- My biggest takeaway is probably that self-governance efforts seem more likely to occur when risks are somewhat prominent. As a result, we could do more to connect “near-term” issues like data privacy and algorithmic bias with “long-term” concerns. We could try to preemptively identify “fire alarms” for TAI, and be ready to take advantage of these warning signals if they occur.
Introduction
Private actors play an important role in AI governance. Several companies have released their own guidelines, and the Partnership on AI is a notable actor in the space.[1] In other words, we are beginning to see elements of self-governance in the AI industry. Self-governance occurs when private actors coordinate to address issues that are not obviously related to profit, with minimal involvement from governments and standards bodies.[2]
Will more substantial self-governance efforts occur? One way to shed light on this question is by looking for previous cases of self-governance to reduce technology risk. In particular, I ask RQ1: Have similar self-governance efforts occurred? I find 6 cases worth further investigation. From the 6 cases, I ask RQ2: What factors make self-governance efforts more likely to occur?
RQ1: Have similar self-governance efforts occurred?
Method
Features of similar efforts
To find out if similar self-governance efforts have occurred, we must first decide what counts as a similar effort. I used the following criteria to evaluate the relevance of past efforts because they seem like important features of AI development today.[3]
- Private: Academic communities or corporate research groups are the leading actors, with minimal involvement from governments or established standards bodies. This is part of the definition of being a self-governance effort.
- Negative externalities: Actors are trying to address issues that affect third parties. For example, issues involving national security or public safety. These risks are not directly related to profit and are traditionally left to governments.
- Involves technology: Companies coordinate on the development or governance of some technology, which can be differentiated from coordinating on other types of products and resources, like forests or food.
Long-listing cases
I generated 20 leads by searching the internet, skimming the literature on self-governance, and asking people at the CLR. Of the resources I found, I referred most to Self-Governance in Science (Maurer 2020). I also posted questions on the EA forum and LessWrong, but these returned few answers.
I spent 1-2 hours reviewing each effort, and rated them high (5), moderate (3), or low (1) on each of the three criteria. These ratings are uncertain and highly subjective, as in natural for short reviews of complex topics. A composite score was calculated by taking a weighted average.[4]
I tried to focus on specific, concrete efforts within a given industry. Sometimes this is fairly clear-cut, as in the case of Leo Szilard. But in other cases, e.g. biology journals trying to restrict publication of dual-use research, it was not obvious if different bursts of activity should be considered one effort or many. Looking for specific efforts has probably caused me to overlook industries where self-governance has come about gradually, but which are nonetheless valuable to study.
Results
The table below shows my ratings. Based on the ratings and my intuitions, I think the first 7 cases are somewhat similar to AI development. Of the 7, I found moderate to detailed information about all except the creation of the 1962 Air Commerce Act, so I excluded this case from further analysis. The spreadsheet of rankings can be found here, and short descriptions of the shortlisted cases are included in the next subsection.
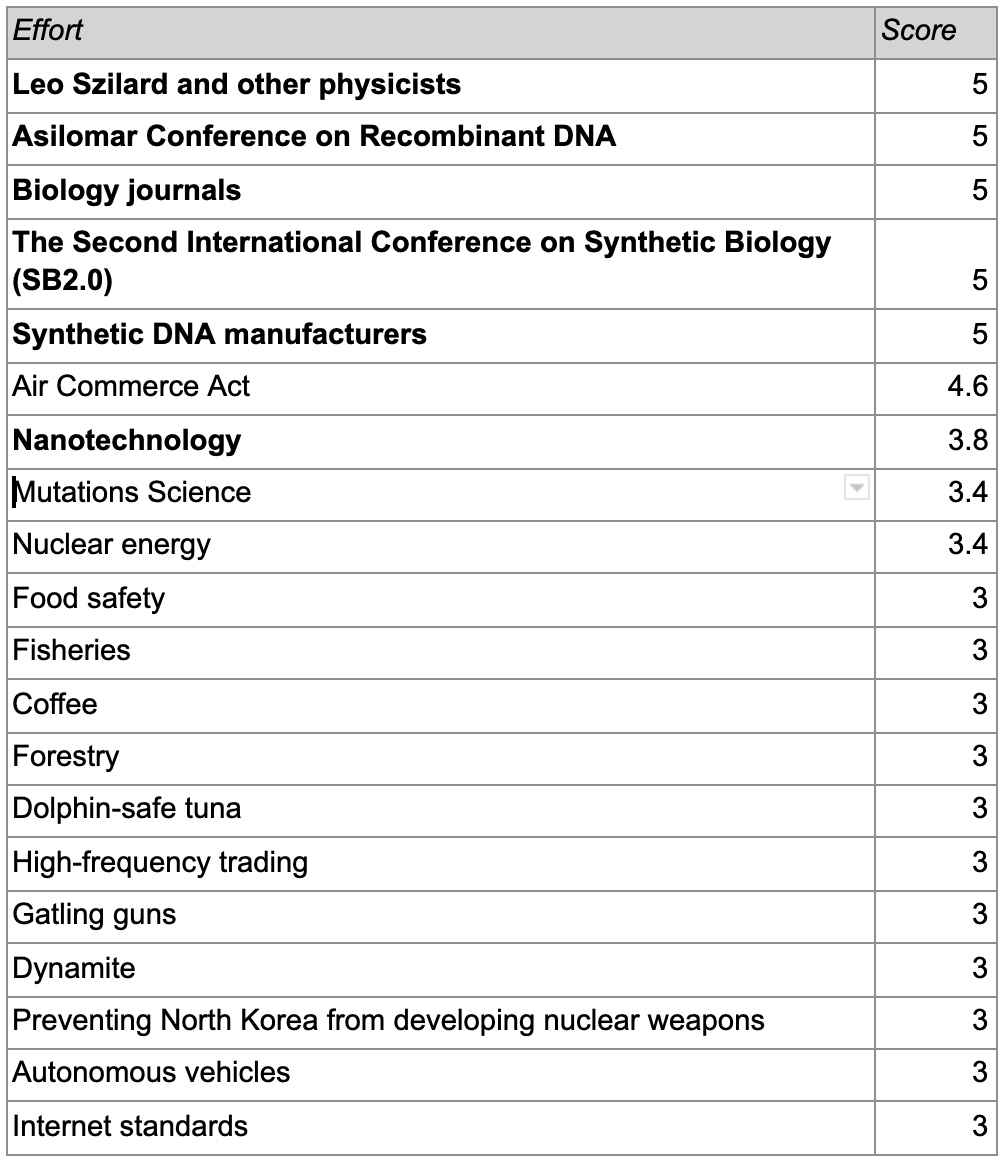
Discussion
Self-governance efforts to manage technology risk are rare. This is mostly because powerful dual-use technologies are rare to begin with, and many of these technologies (e.g. nuclear power, cryptography) were developed by governments.
Of the six efforts that seem relevant to AI development and that I found at least moderate information on, the two that were most successful are the actions by Leo Szilard and other physicists from 1939–1940 to prevent nuclear research from proliferating to Germany, and the 1975 Asilomar Conference on Recombinant DNA. These cases are already well-known, and within the EA community they have already received detailed treatment by AI Impacts and MIRI.[5]
All six efforts are summarised in the table below.
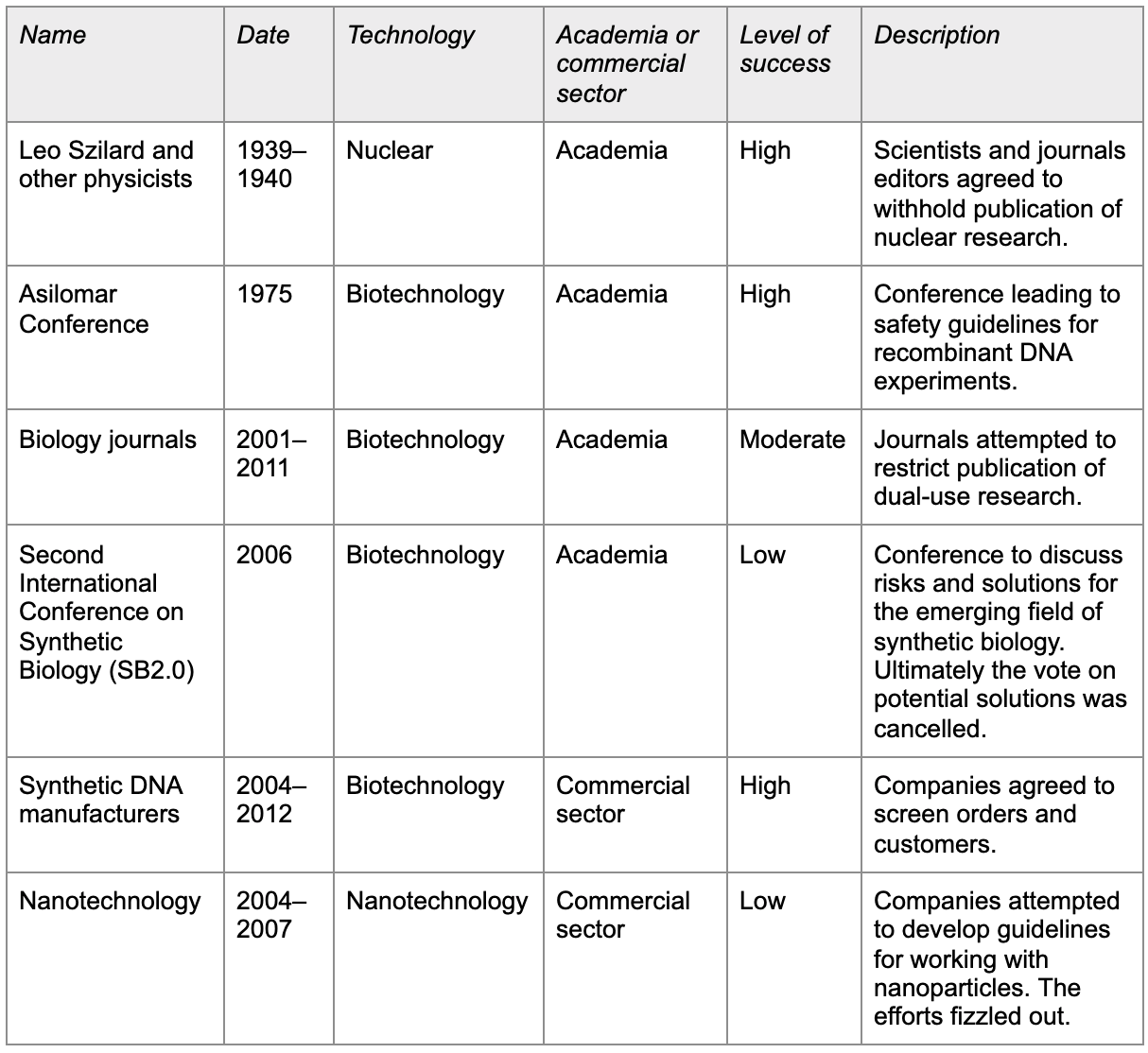
RQ2: What factors make self-governance efforts more likely to occur?
Given the complexity of each case, I find it hard to tease out specific factors that apply across cases, and determine how much each factor matters. The small number of cases, and the fact that many of them come from one sector (biotechnology), makes me uncertain about the generalisability of these insights. I am not a historian and am wary of telling chronological narratives and assuming causal relationships.
With these limitations in mind, here are some factors that seem to make self-governance efforts more likely to occur. No single factor is decisive, and the most successful efforts exhibited all or almost all the factors to a high degree.
Risks and threats are salient
In the successful cases, events occurred that made the technology risk seem plausible. Szilard’s efforts succeeded as the war and the threat of a German nuclear advantage became more serious. Asilomar took place around the time the Biological Weapons Convention created a strong international norm against bioweapons, and after potential hazardous experimental results about recombinant DNA were produced by Paul Berg and others. September 11 and the anthrax attacks significantly increased public and government awareness about bioterrorism, and subsequent bursts of activity were usually a reaction to high-profile dual-use experiments. Conversely, where this feature was relatively absent (nanotechnology), self-governance efforts were unsuccessful.
This might suggest that we should be pessimistic about catalyzing self-governance efforts via abstract arguments and faraway failure modes only. We could do more to connect “near-term” issues like data privacy and algorithmic bias with “long-term” concerns. We could try to preemptively identify “fire alarms” for TAI, and be ready to take advantage of these warning signals if they occur.
“Shadow of hierarchy”
Government actions and the threat of further (poorly-designed) regulation can spur private actors to govern themselves. A large motive of the Asilomar Conference was to avoid regulation of recombinant DNA technology by outsiders. After the 2001 anthrax attacks, regulations like the PATRIOT ACT, Bioterrorism Preparedness Act, and the 2004 Fink report suggested that the US government might take further action to regulate biotechnology. These initiatives arguably played a role in encouraging attempts at self-governance by biology journals, the synthetic biology community, and synthetic DNA manufacturers. Note, however, that the shadow of hierarchy appears to be absent in the case of Leo Szilard.
This factor suggests that the notion of “pure” self-governance, involving almost no involvement from other types of actors, seems mistaken. In the real world, governance involves multiple interacting efforts from private actors, standards bodies, governments, and more.
Field or industry is small
According to Martin Rees, the small size of the field of recombinant DNA in the Asilomar days made voluntary consensus more achievable.[6] The same might be said for the physics community in 1939; Szilard was able to contact many influential scientists personally. In the synthetic DNA manufacturing industry, 4 companies controlled 80% of the market.
This factor increases the importance of acting sooner rather than later, while the relevant communities are smaller. It seems plausible that the AI community, broadly defined, is big enough to make self-governance much harder. However, the relevant community might be groups attempting to build AGI, in which case prospects for self-governance are more promising.
Support from gatekeepers: journals and anchor firms
Gatekeepers are actors who decide who is “in or out”. Szilard’s campaign succeeded after the National Academy of Sciences, with support from journals, organised a program to filter and withhold sensitive papers. The pre-Asilomar moratorium and the conference itself were suggested by scientists with the help of journals. Journals themselves made various efforts to restrict publication from 2001–2011, with low to moderate success. Where this factor was absent (synthetic biology), the self-governance effort was unsuccessful.
In the commercial sector, downstream anchor firms[7] are one type of gatekeeper. In the case of synthetic DNA manufacturing, large pharmaceutical companies with significant purchasing power put pressure on DNA manufacturers to screen orders and customers. Downstream firms tend to be more visible and answerable to consumers and regulators, and thus more likely to encourage higher safety standards for PR reasons.
Applied to AI governance, this factor suggests that ongoing efforts to shape norms through journals and conferences are a promising move. As one might expect, engaging with large technology companies seems important.
Support from credentialed scientists
In Szilard’s case, Fermi’s (reluctant) decision to suppress a new experiment on carbon cross sections is considered to have been one “turning point”. At Asilomar, support from big names like Paul Berg, David Baltimore, Sydney Brenner, Richard Roblin, and Maxine Singer helped to legitimize the recommendations. Note that other well-known scientists like James Watson, Stanley Cohen, and Herbert Boyer criticised the process and voted against the recommendations. This might suggest that the self-governance effort does not need consensus support but at least a few highly credential scientists have to get behind it.
Other observations
Government codifies rules initially developed by private actors
In addition to threatening regulation before self-governance occurs, governments can take action after the initial self-governance effort. After WW2, the US government formalised the restriction of nuclear research through the 1946 “Born Secret” clause. After the Asilomar conference, recommendations from the conference were adopted by the National Institute of Health as mandatory funding requirements. After synthetic DNA companies agreed on standards, the US Department of Health and Human Services (HSS) published its own document.[8]
Summary of RQ2 findings
The table below summarises the degree to which each factor was present in each event:
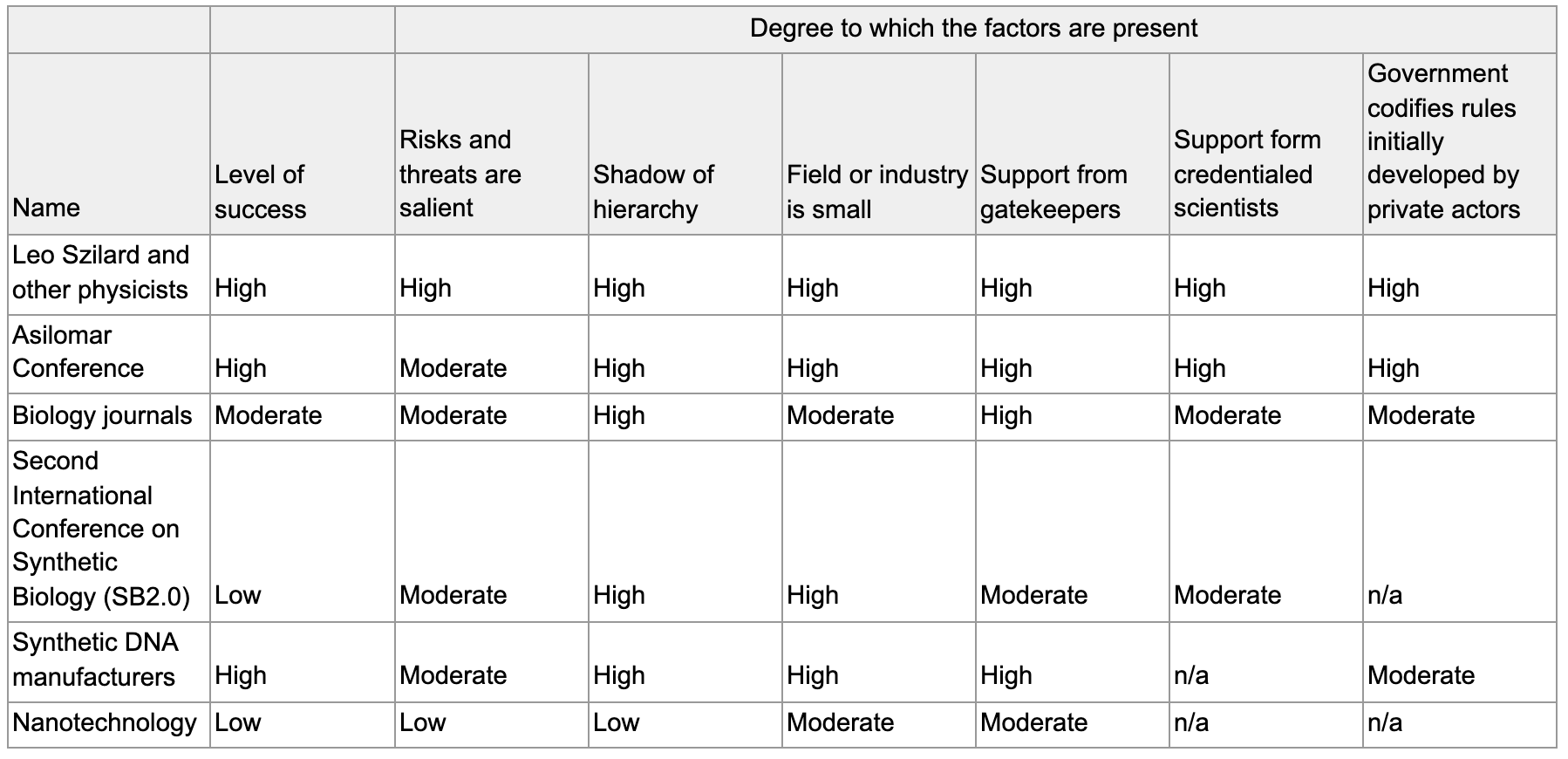
Thanks to everyone at the Center on Long-Term Risk for helpful feedback and conversations, especially Stefan Torges, Mojmír Stehlík, Michael Aird, Daniel Kokotajlo, and Hadrien Pouget. And thanks to Linh Chi Ngyuen for managing the summer research fellowship!
Notes
Gijs Leenders, ‘The Regulation of Artificial Intelligence — A Case Study of the Partnership on AI’, Medium, 23 April 2019, https://becominghuman.ai/the-regulation-of-artificial-intelligence-a-case-study-of-the-partnership-on-ai-c1c22526c19f. ↩︎
Stephen M. Maurer and Sebastian von Engelhardt, ‘Industry Self-Governance: A New Way to Manage Dangerous Technologies’, Bulletin of the Atomic Scientists 69, no. 3 (1 May 2013): 53–62, https://doi.org/10.1177/0096340213486126. ↩︎
I initially wanted to score efforts according to two additional features that seem relevant to AI governance: “conflicting interests” and “complexity”. However, I lacked sufficient information to make an assessment after 1 hour of research per historical case of self-governance efforts, so ultimately I excluded these features. Conflicting interests means that actors have to pay different costs in terms of time and money, and prefer different outcomes; the situation is not a pure coordination game. Complexity means that the risks involved are uncertain and there is no clear consensus on solutions. ↩︎
“Private” and “involves technology” were weighted 2, while “negative externalities” was weighted 1. ↩︎
Preliminary survey of prescient actions; The Asilomar Conference: A Case Study in Risk Mitigation; Leó Szilárd and the Danger of Nuclear Weapons: A Case Study in Risk Mitigation. ↩︎
“There are now even more reasons for exercising restraint, but a voluntary consensus would be far harder to achieve today: the community is far larger, and competition (enhanced by commercial pressures) is more intense.” Rees, Martin J. Our Final Hour: A Scientist’s Warning: How Terror, Error, and Environmental Disaster Threaten Humankind’s Future in This Century on Earth and Beyond. New York: Basic Books, 2004. ↩︎
Anchor firms are firms that have an influential position in the market. ↩︎
Strangely, however, the document was weaker than either of the private standards. ↩︎
Thanks for this post!
Four things I particularly liked about this post were that:
Planned summary for the Alignment Newsletter:
Cool post! I like the methodology; it bears a lot of similarities to the case studies summary and analysis I'm writing for Sentience Institute at the moment. What do you think about the idea of converting those low / moderate / high ratings in the RQ2 table into numerical scores (e.g. out of 5, 10, or 100) and testing for statistically significant correlations between various scores and the "level of success" score?
This seems like a reasonable takeaway from these case studies. But it seems like the case studies mainly demonstrate that the salience and perceived plausibility of a technological risk is important, rather than that the best method for increasing the salience and perceived plausibility of a technological risk is to connect long-term/extreme risks to near-term/smaller-scale concerns or to identify "fire alarms"?
Perhaps making people think and care more about the long-term/extreme risks themselves - or the long-term future more broadly, or extinction, or suffering risks, or whatever - could also do a decent job of increasing the salience and perceived plausibility of the long-term/extreme risks? I'd guess that it's harder, but maybe that disadvantage is made up for by the fact that then any self-governance efforts that do occur would be better targeted at the long-term/extreme risks specifically?
Also, the nuclear and bio case studies involved technological risks that are more extreme or at least more violent and dramatic than issues like data privacy or algorithmic bias. So it actually seems like maybe the case studies would push against a focus on those sorts of issues, and in favour of a focus on the more extreme/violent/dramatic aspects of AI risk?
Maybe some parts of what you read that didn't make it into this post seem to more clearly push in favour of connecting "long-term" AI concerns to "near-term" AI concerns?
Thank you for this post, I thought it was valuable. I'd just like to flag that regarding your recommendation, "we could do more to connect “near-term” issues like data privacy and algorithmic bias with “long-term” concerns" - I think this is good if done in the right way, but can also be bad if done in the wrong way. More specifically, insofar as near-term and long-term concerns are similar (eg., lack of transparency in deep learning means that we can't tell if parole systems today are using proxies we don't want, and plausibly could mean that we won't know the goals of superintelligent systems in the future), then it makes sense to highlight these similarities. On the other hand, insofar as the concerns aren't the same, statements that gloss over the differences (eg., statements about how we need UBI because automation will lead to super intelligent robots that aren't aligned with human interests) can be harmful, for several reasons: people who understand the logic doesn't necessarily flow through will be turned off, if people are convinced that long-term concerns are just near-term concerns at a larger scale then they might ignore solving problems necessary for long-term success that don't have near-term analogues, etc.
I just stumbled upon the 2013 MIRI post How well will policy-makers handle AGI? (initial findings), found it interesting, and was reminded of this post.
Essentially, the similarity is that that post was also about AI governance/strategy, involved thinking about what criteria would make a historical case study relevant to the AI governance/strategy question under consideration, and involved a set of relatively shallow investigations of possibly relevant case studies. This seems like a cool method, and I'd be excited to see more things like this. (Though of course our collective research hours are limited, and I'm not necessarily saying we should deprioritise other things to prioritise this sort of work.)
Here's the opening section of that post: