In this EA Student Summit 2020 talk, Amanda Ngo shares reasons to be excited about forecasting, walks through a process to forecast questions you care about, and shows how Ought's tool, Elicit, can be helpful for this.
Below is a transcript, which we've lightly edited for clarity.
The Talk
Habiba Islam [00:00:00]: Hello, and welcome to this session on estimation and forecasting with Amanda Ngo. I'm Habiba and I'll be the emcee for this session. We'll start with a 15-minute talk by Amanda, then we'll move on to a live Q&A session where she'll respond to some of your questions. You can submit questions using the box to the right hand side of the video. You can also vote for your favorite questions to push them higher up the list.
Now, I'd like to introduce you to the speaker for the session. Amanda works on forecasting tools at Ought. She graduated from the Huntsman Program in International Studies and Business at the University of Pennsylvania, where she worked with Dr. Angela Duckworth on self-control research. She previously interned in growth marketing, software, engineering, and journalism. Here is Amanda.
Amanda Ngo [00:00:47]: Hi, everyone. My name is Amanda. I'm excited to chat to you today about estimation and forecasting and how to get started with forecasting. I hope that at the end of today you are excited about the value that forecasting can add to your life, and you also feel confident that you have a roadmap to get started.
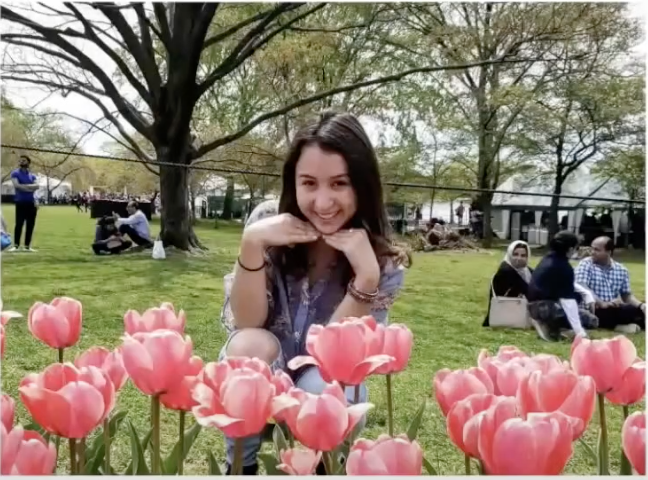
This is a photo of me in college. I now work at the research nonprofit Ought doing business operations and product work.
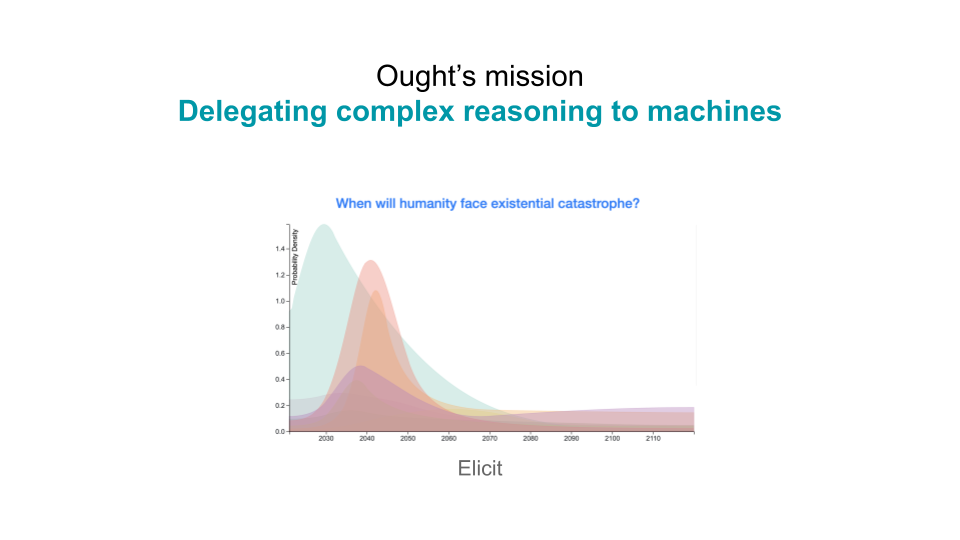
Ought's overall mission is to delegate complex reasoning to machines, so there are a lot of questions that we care about — for example, when will humanity face existential catastrophe? These are complex questions. It's hard to know if you have good answers to them, but we still care a lot about finding some.
[00:01:35] Ought is working on how to make machine learning as useful as possible for answering these kinds of questions. By default, machine learning is not as useful when a question is not clearly defined, when there's not a lot of training data, when there isn't a clear reward signal, or when it's hard to tell whether you've generated a good answer. And it's not clear that as machine learning scales, it's going to get better at this kind of complex reasoning in a way that we can trust.
[00:02:04] One approach to address this, which Ought is doing, is to break down the reasoning process into smaller components that you can automate, and whose performance you can evaluate. This is a more incremental approach, where we're building a system that can help humans reason better, and as machine learning improves, we can plug that in.
Elicit is the first step on this journey. Currently, Elicit lets you enter in beliefs that you have about any forecasting question. It will automatically create a probability distribution that's consistent and captures those beliefs as well as possible. And in the future, Elicit will automate more and more of the reasoning process. So, for example, it might suggest factors that are useful for you to consider based on language model suggestions.
[00:02:56] This is why we're excited about forecasting. But why should you care about applying forecasting to your own life?
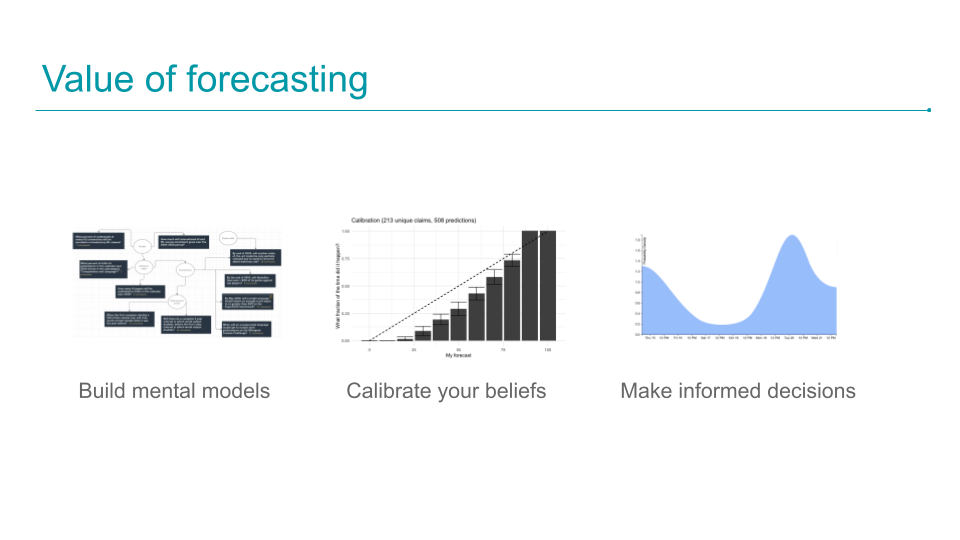
There are several benefits that we found from doing a lot of forecasting ourselves:
- Forecasting is a forcing function for building mental models about the world. Trying to assign numbers to a question really forces you to think through the causal factors that would make an event happen. What would have to be true for this event to happen? What information in the world would update me, one way or the other? [00:03:28] For example, we facilitated a set of predictions on artificial general intelligence (AGI) timelines on LessWrong, and what we found from going through that process was that it crystallized the models that people were using about how we might reach AGI — for example, the probability that we'd get AGI from language models and the probability that we'd need a paradigm shift.
- [00:03:51] Forecasting can help you calibrate your beliefs. What forecasting really does is require you to say, “I have a belief. Let's test it against the world and see if it's accurate.” We do a lot of project timeline estimation at Ought, and what we found is that when people do project timeline estimations, we're consistently overconfident. Tracking these predictions allows us to use that knowledge to adjust downwards.
- [00:04:18] Forecasting can help you make more informed decisions. This refers to decisions at two levels. First, if you have better predictions for AGI timelines, you can make better career decisions about whether to go into AI safety. And second, on the level of project timelines, you can plan initiatives if you have better estimations of when you might launch a feature.
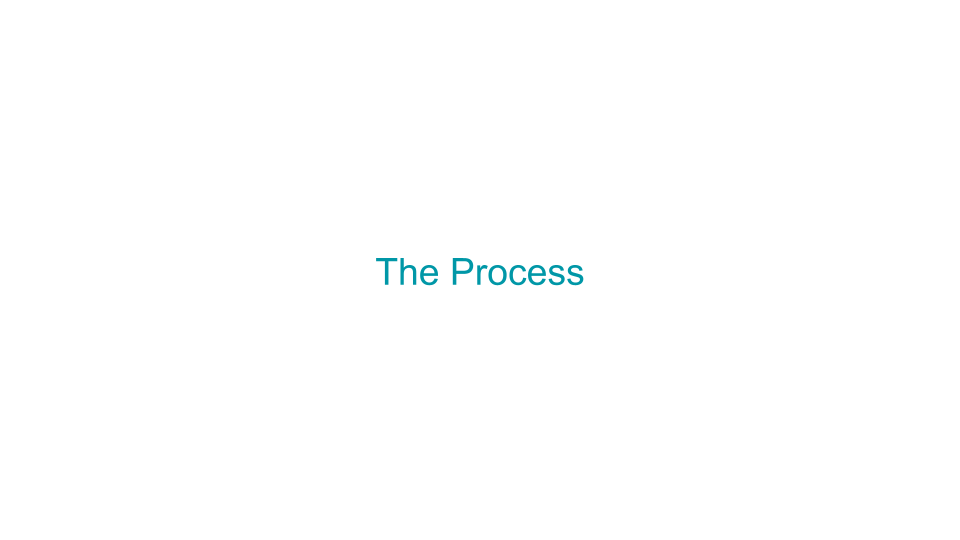
[00:04:40] Now let's jump into a process that you can use to actually make forecasts. It's worth noting here that forecasting is a pretty nascent field. There's a lot of debate and discussion about what the different best practices are and which paradigms to use, and every forecaster has a slightly different process. I'm going to point out a few tools that are helpful, but it's worth keeping in mind that this isn't comprehensive.
[00:05:12] The first stage is to pick the question that you're going to forecast. It's worth spending a fair amount of time on this because you want to make sure that the question actually captures what you care about and is measurable. There has to be a metric that allows you to tell whether the answer to your question is true or false. You might start with a vague question like “Should I do a master's degree?” We can't forecast this yet because there's no outcome.
You would need to narrow it down into a question like “How useful will it be for my career?” Now you have an outcome: its usefulness. But you still don't have a metric that can tell you how useful it actually was.
[00:05:55] To get to the third level, which is generating measurable questions, there are often a lot of metrics that we can use for any outcome or vague question. In this case, a few examples are “How many LinkedIn connections will I have as a result of my master's degree?” and “How many of my top 10 companies will give me a job offer if I do a master's degree?”
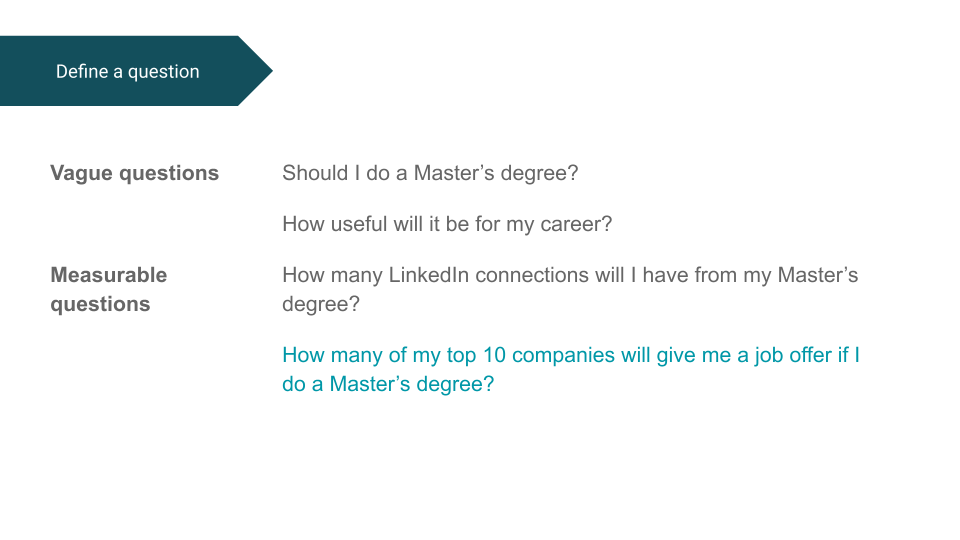
You want to forecast based on a cluster of these, but we're going to zoom in on “How many of my top 10 companies will give me a job offer?”
[00:06:26] Now you can make the forecast. The first key is to start with the outside view, which indicates that the event you're forecasting is going to look like similar events in the past. Basically, what you want to do is find what we call a reference class.
A reference class is a set of events that behaves similarly. You can use it to extrapolate in making your current forecast. We're often overconfident about how the event that we're forecasting is going to be different; this is a classic planning fallacy. When you're planning how long a project is going to take, you will likely be overconfident. Therefore, it's better for you to just look at how long similar projects took in the past.
It takes some skill to figure out which reference class to use. There are often multiple options and you have to choose the one that’s most useful for the question you're forecasting. In this case, some reference classes might be how many job offers people with master's degrees who applied to similar companies received, or how many job offers you received out of undergrad.
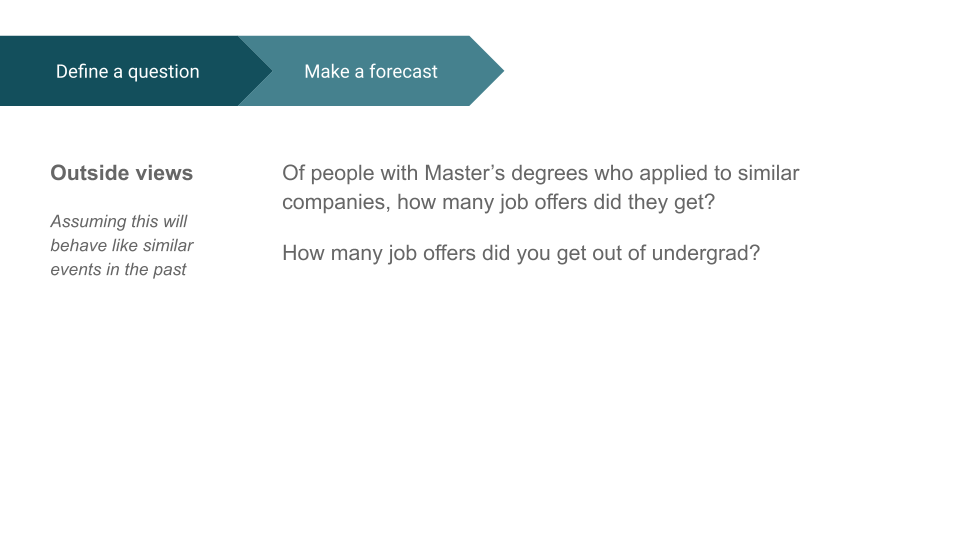
[00:07:36] Once you have your outside view, you can then adjust it based on what we call an inside view. This is more about building an actual model of the event that you can use to be more specific than just your extrapolation of the outside view. There are a lot of different ways to approach this. I'm going to outline a few, but there are many more, and often you want to use multiple angles to see if they align and produce the same answer.
[00:08:06] One angle is to consider factors that would lead to the outcome. When forecasting job offers, your GPA is going to influence the outcome, the state of the economy is going to influence how many jobs are available, and how good your research papers are might influence your chances of getting a job. You can go even further up the causal chain to forecast which factors would lead to you producing good research.
[00:08:33] A second way to think about it is to generate sub-questions. For example, you could break this question down into:
- What's the probability that I get a job offer from each company? Then, you could combine those answers to get your overall forecast.
- Will I get an internship offer from one of my top 10 companies? And what's the probability that this will convert through to a full-time offer? You could also break that down into scenarios.
This approach is helpful for making sure that you have comprehensively covered what might happen, even at the extreme edges. So you might think, “Well, there's a possibility that I won't graduate, and maybe then I'll get zero jobs. And maybe the likelihood of that is 1%.” There's also the possibility that you pivot your degree entirely, and maybe that leads to a lot more job offers. Perhaps the likelihood of that is 10%. There are many more possibilities as well.
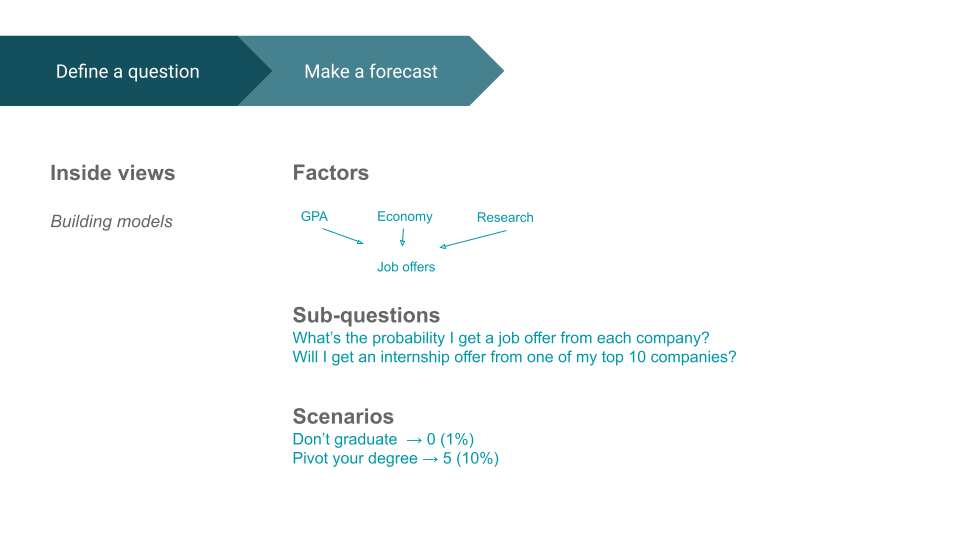
[00:09:25] Now that you have your forecast, you want to sense-check it to make sure you really believe it. The first thing you can do is examine the implications of your belief. So you might want to ask yourself, “Would I bet on this?” We find that when we ask that, we discover that we’re overconfident and not willing to actually make a bet on the forecast that we've made.
[00:09:48] Second, you can sense-check the summary statistics from the forecast. See what the median and the mode are: the twenty-fifth and seventy-fifth percentile, and if those seem reasonable. You can also look at related questions. If you previously forecasted your probability of getting an internship, then that might be related to the probability that you get a job. You’d want to check to see if those align and seem reasonable in terms of how they relate.
[00:10:20] Then you can compare your forecasts. We find it really useful to send out forecasts to each other. We compare them and see where we disagree the most — and, crucially, find which factors and assumptions make us disagree the most.
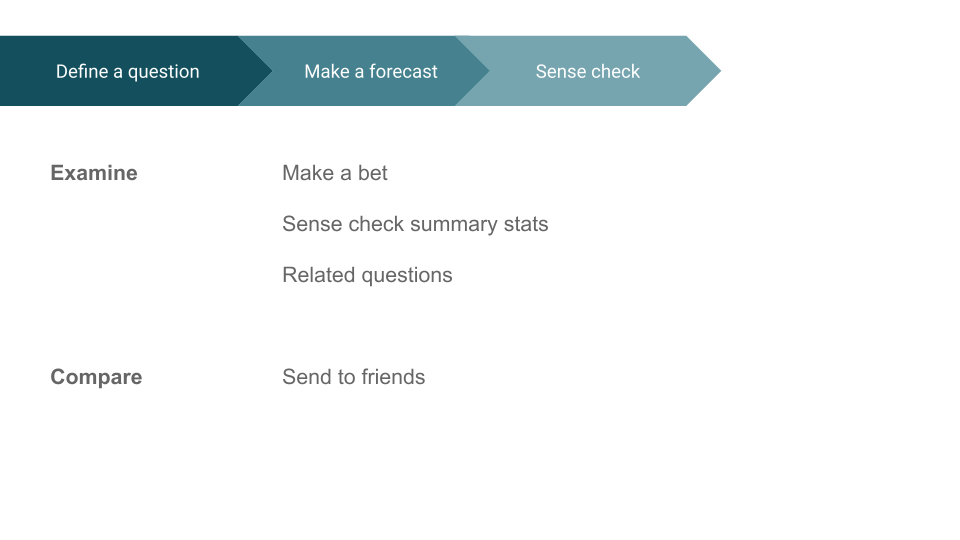
You’ll want to revisit your forecast. It's key to update it frequently. As new information comes in, your forecast will change. So you might come back and update your forecast if the economy improves, or if you get an internship offer. Also, track your forecasts so you can calibrate and learn over time.
[00:11:00] You actually can't tell how accurate you were based on one forecast alone. Let’s say you forecasted an 80% chance that you’d get a job and then you don't. You can't tell if it was because you made a bad forecast or because you were in the 20% zone.
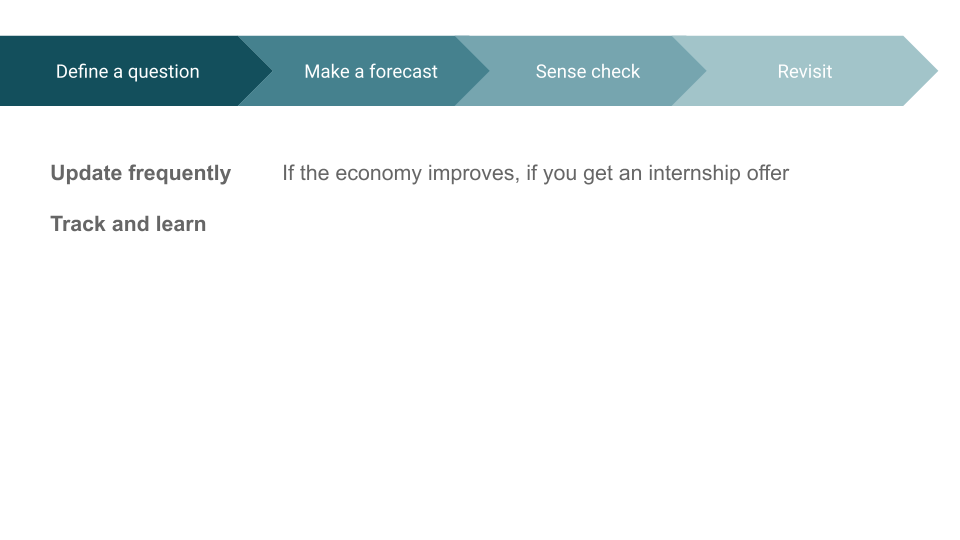
But if you track a lot of forecasts, when you say you're 80% confident on a question, those results should happen 80% of the time. You’ll also learn whether you're overconfident or underconfident in certain domains.
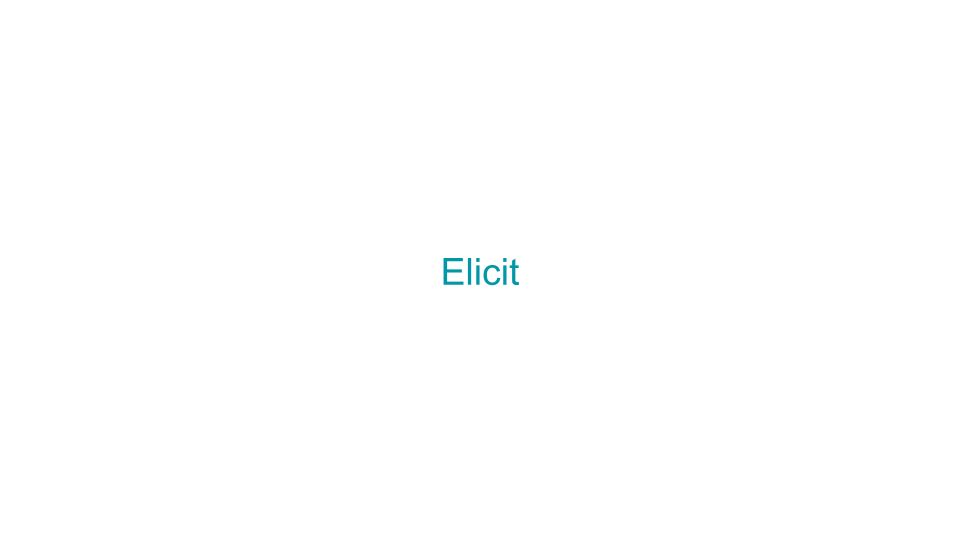
[00:11:40] Now we're going to do a walkthrough of Elicit. I’ll show you how it can be used for the question that we just forecasted.
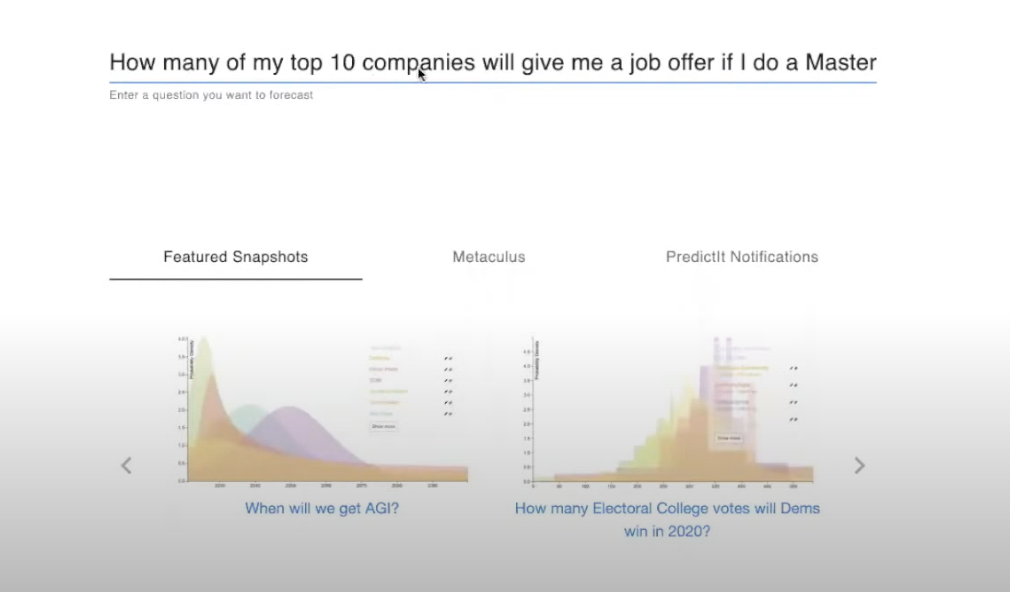
[00:11:49] Here is the Elicit homepage with the question we were forecasting before: “How many of my top 10 companies will give me a job offer if I do a master's degree?”
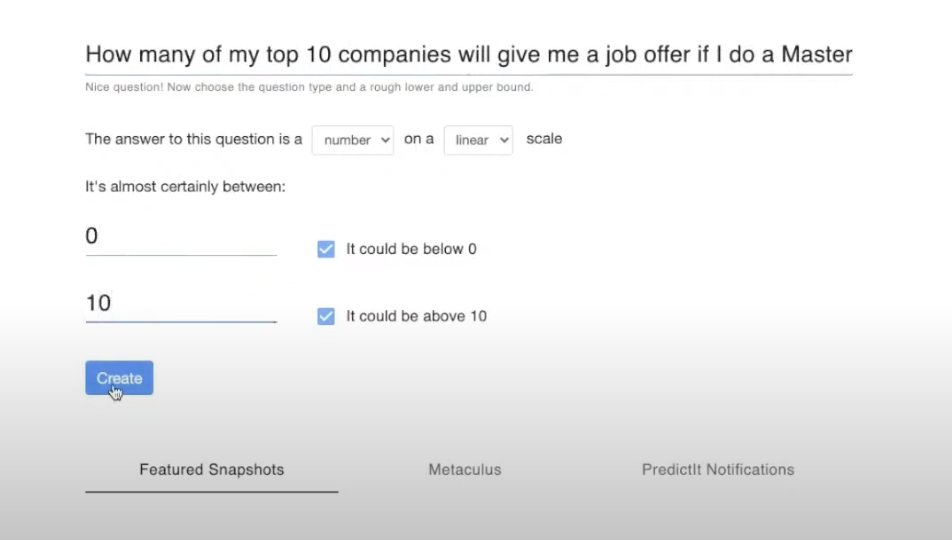
The first thing Elicit asks you to do is identify a lower and upper bound for your probability distribution (i.e., where you think most of the probability falls). In this case, it's easy. We've already said it has to be between zero and 10. So we enter that and click “create”.
This takes you to the question page. Here you have a probability distribution and a place to enter in your beliefs. We're going to follow the process that we just outlined.
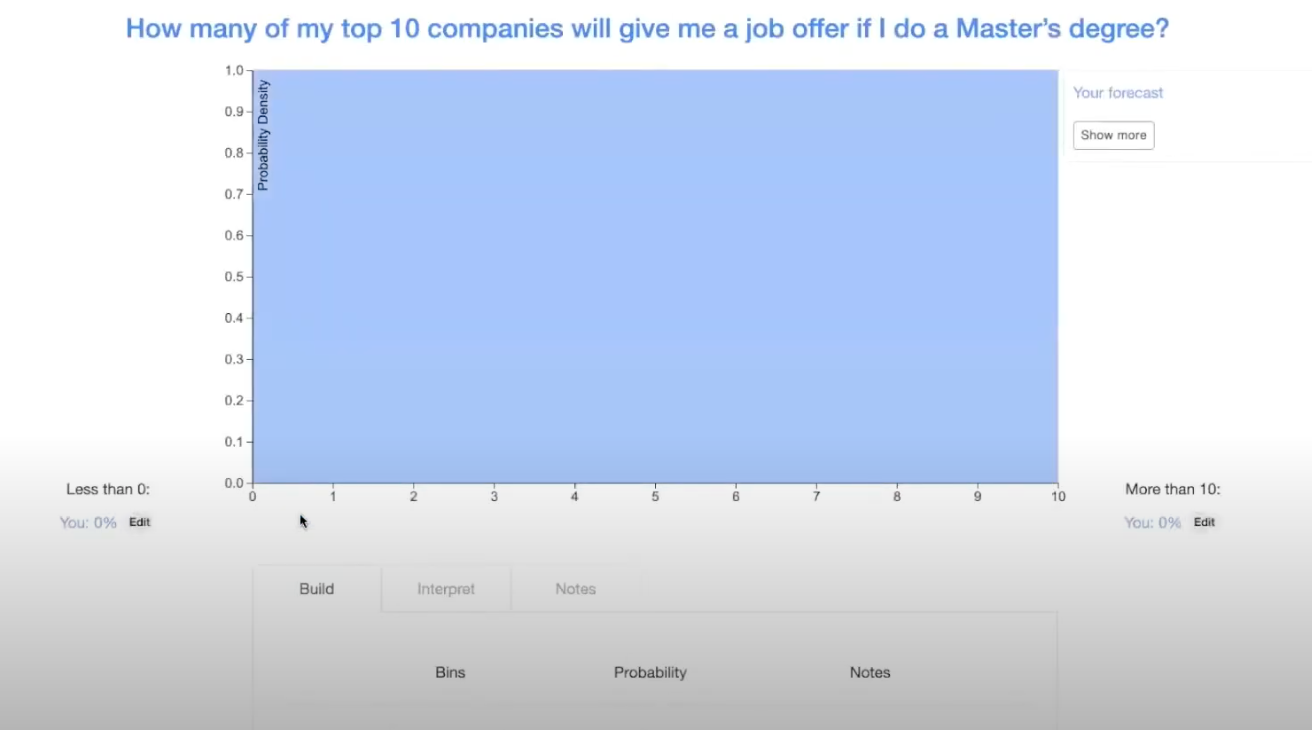
[00:12:26] We start with the outside view.
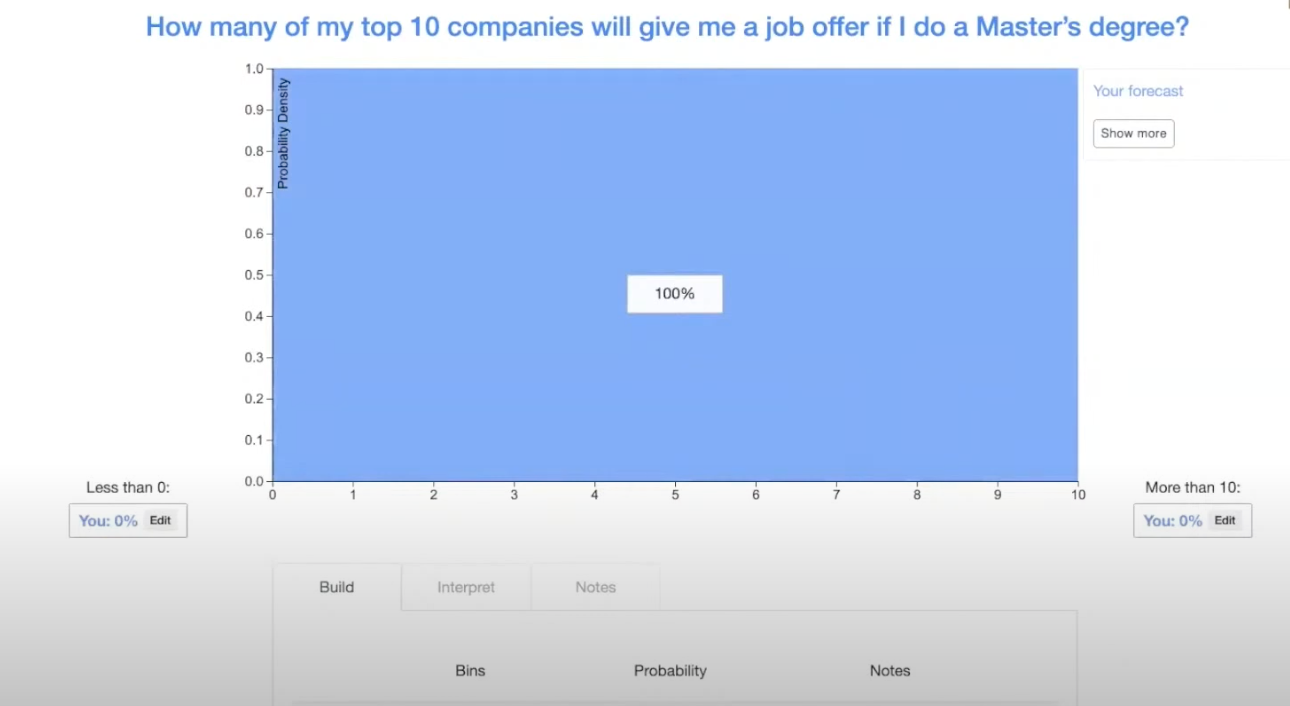
Say you talked to several previous graduates of the master's degree program who had applied to similar companies, and, on average, they received between one and three job offers. This might be a reasonable place for you to start.
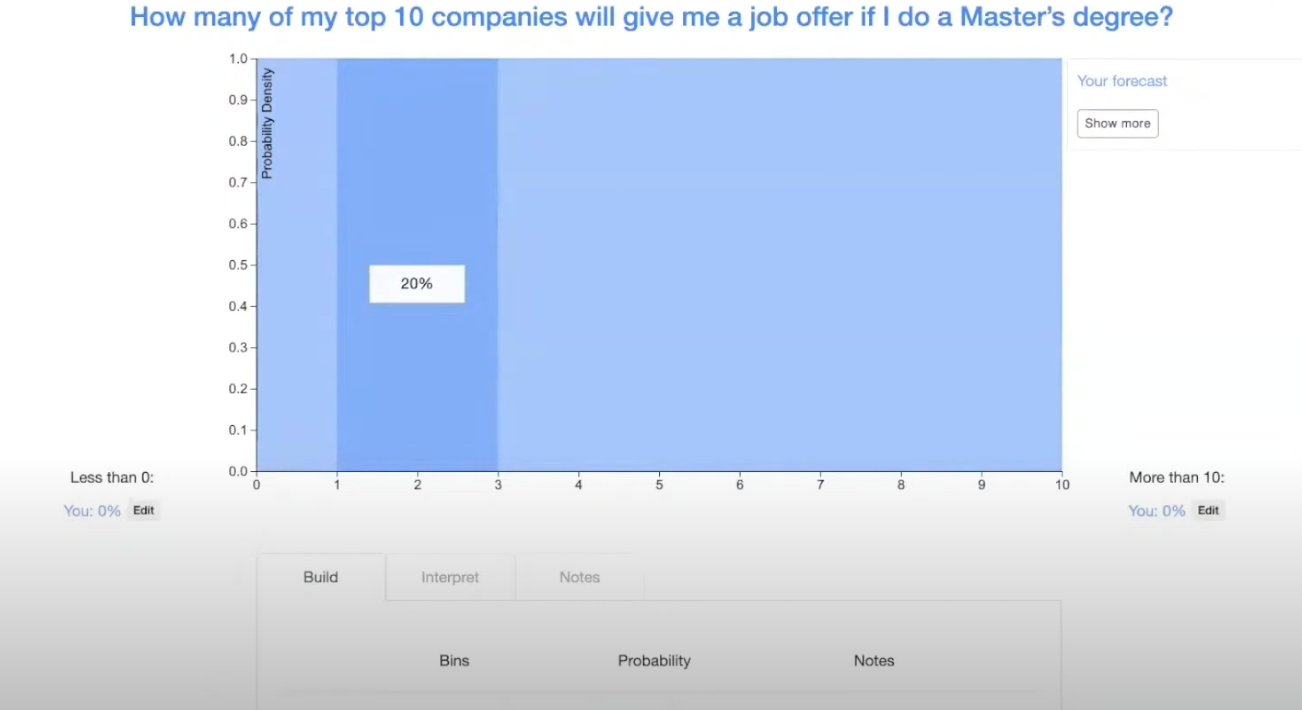
And then you have some additional information about the specifics of your situation. For example, you know that the economy is worse because of COVID, and how the economy is performing affects how many job offers you get. You might want to increase the probability in the lower range from one to two.
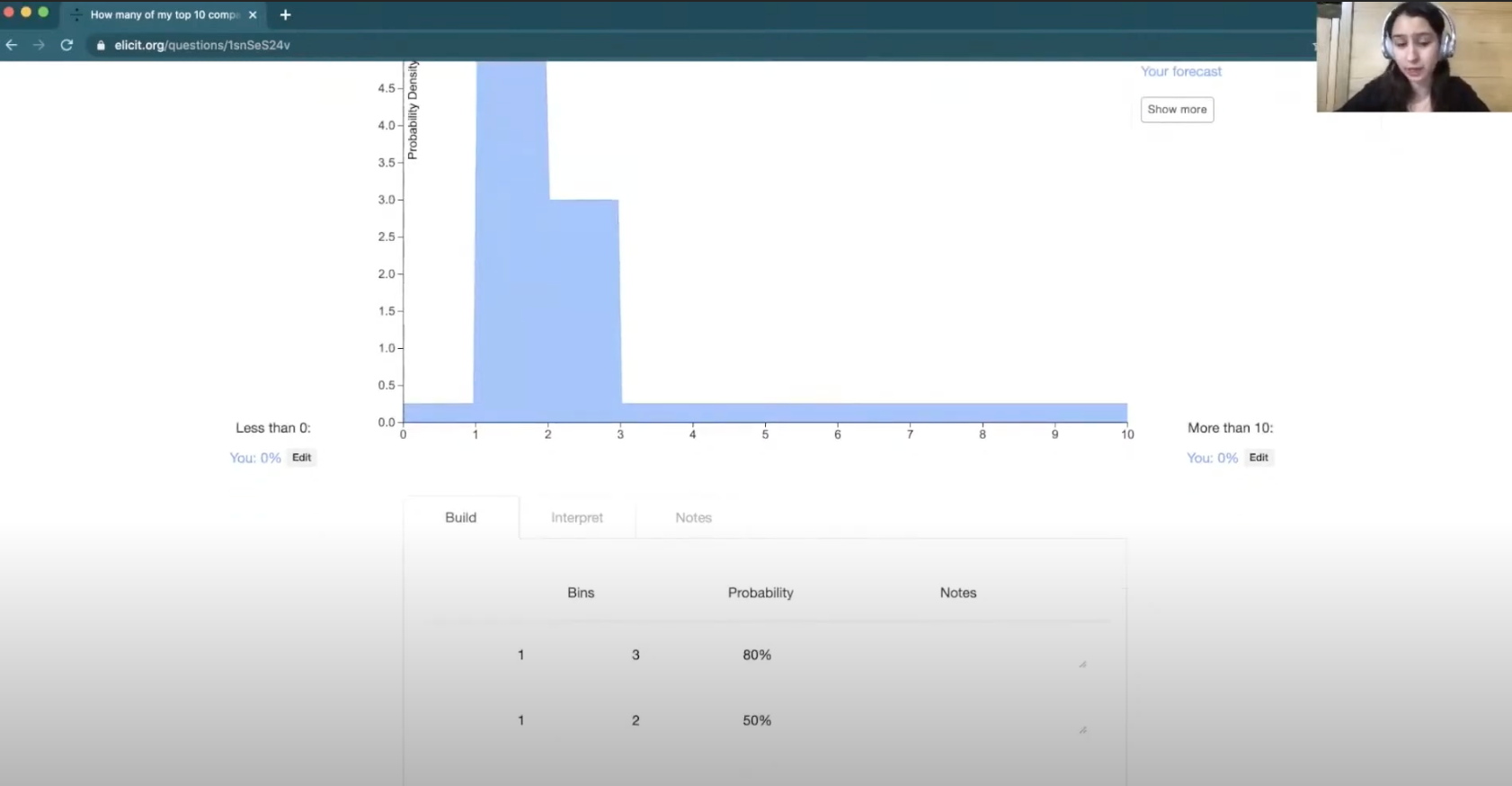
Then, let’s say you're also thinking about different scenarios that could occur and you believe there’s some possibility of pivoting your degree into something that's more hirable, resulting in more job offers. So you might want to use a slightly higher probability in the three-to-four range.
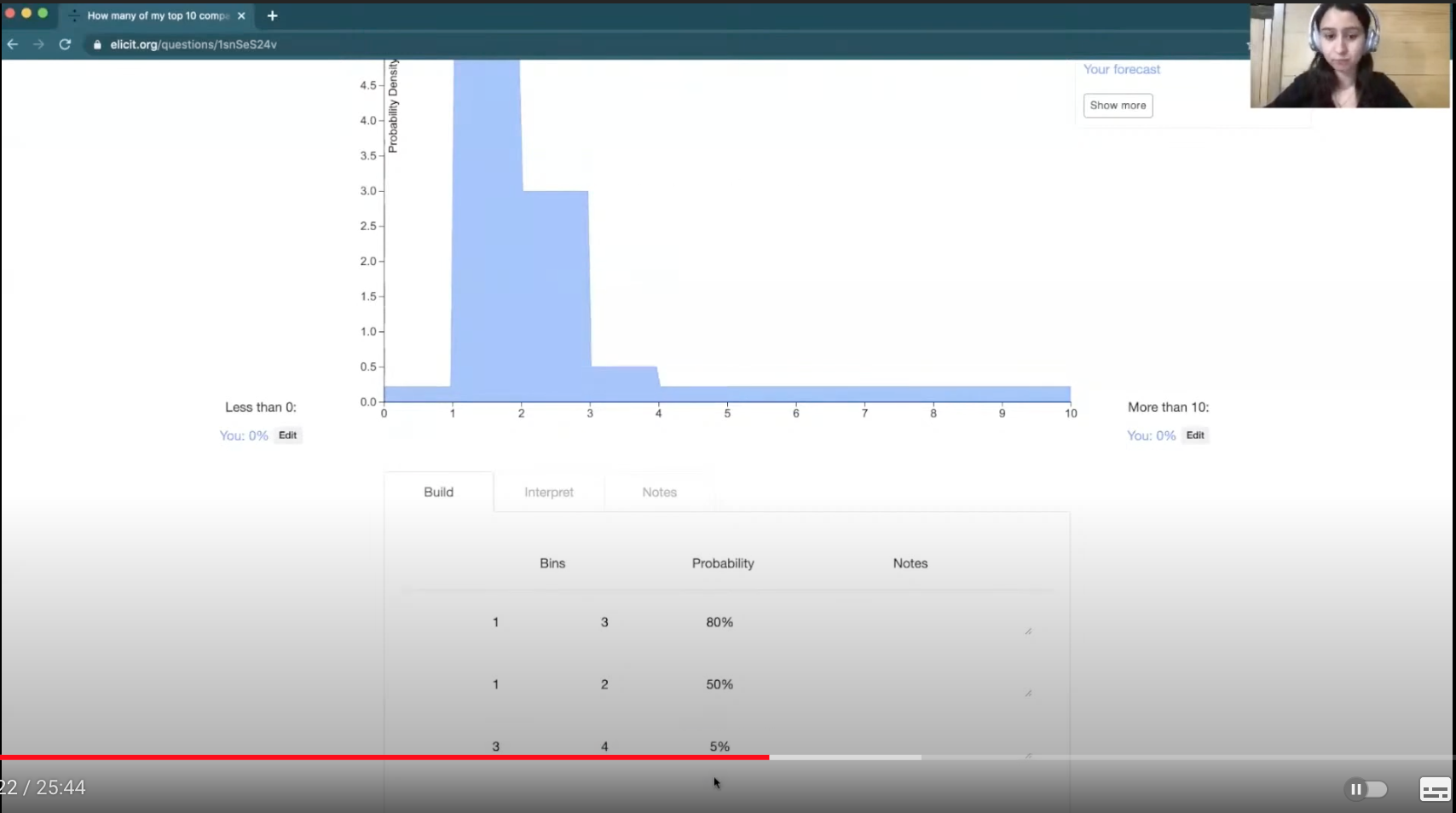
[00:13:22] Next, you can click “smoothing” to generate something that looks more continuous.
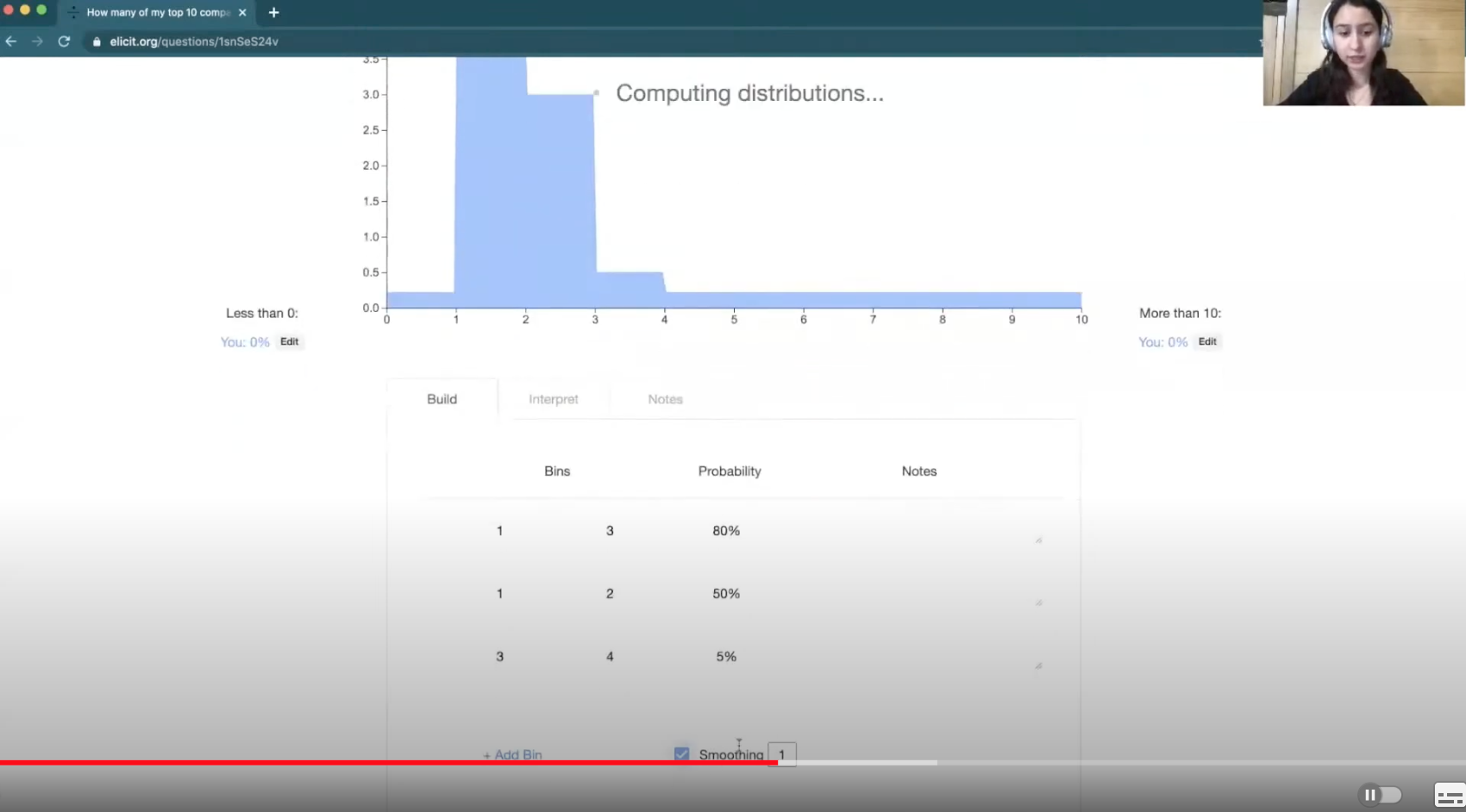
And then you're done with your forecast, so you click “save snapshot” and this will save your forecast in your snapshot history, enabling you to come back and revisit it.
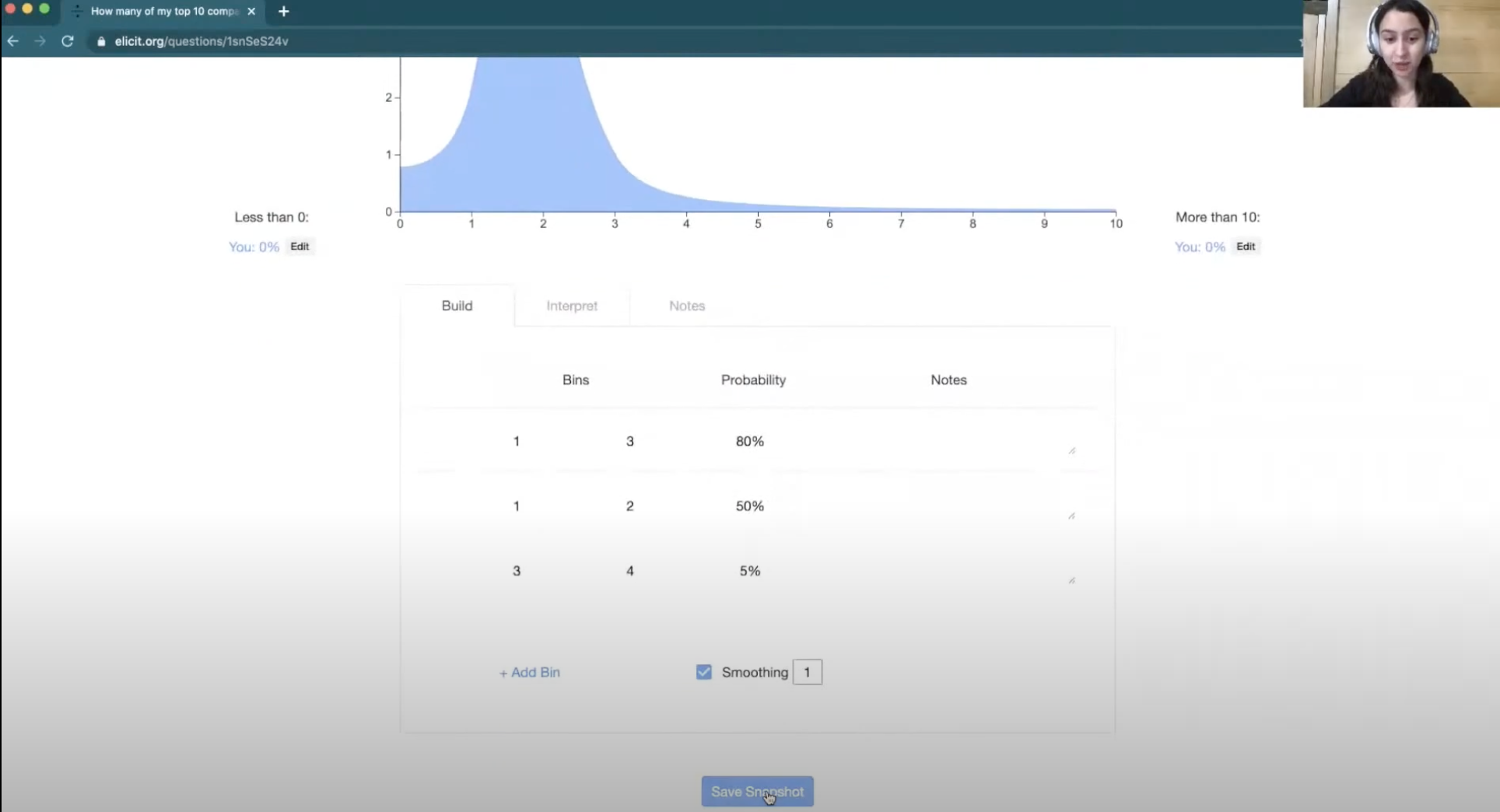
Now we can move on to the sense-checking phase. If you go to the “interpret” tab, it'll tell you some information about what your forecast implies.
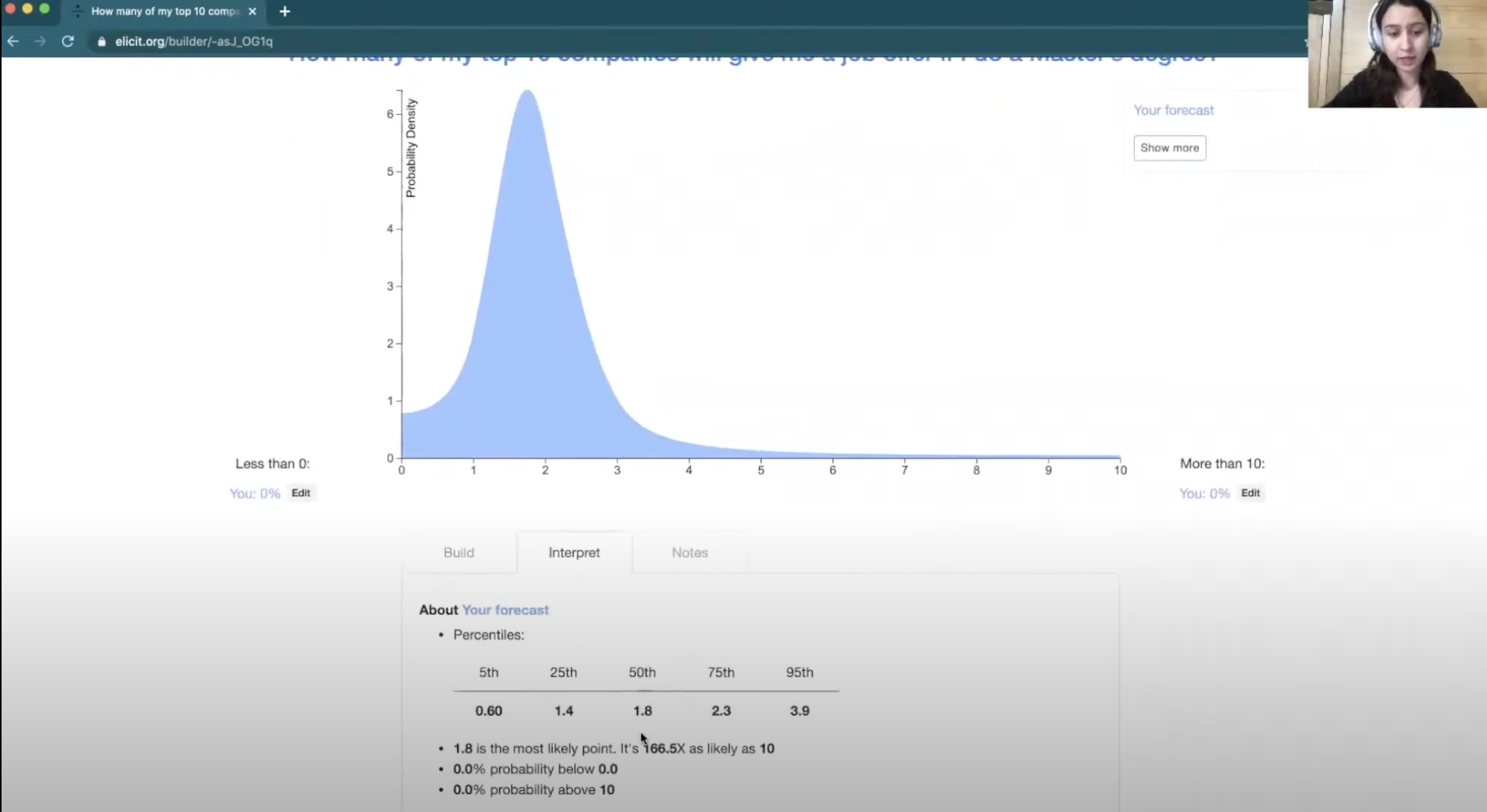
Here you can see the fiftieth percentile, or median, is 1.8. And the most likely point, or the mode, is also 1.8.
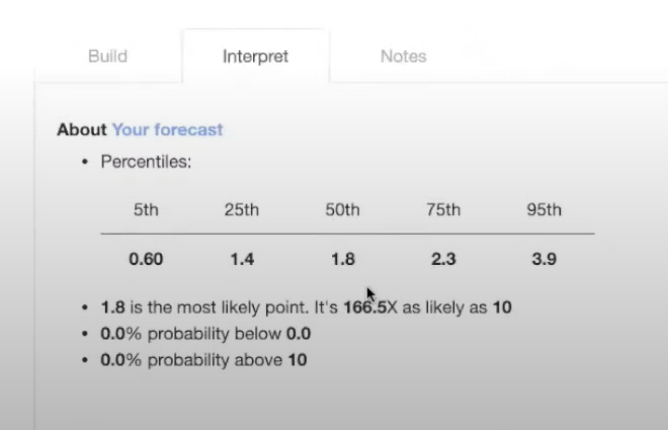
Maybe this sounds a little low to you, so you go back to “build” and decrease this a little.
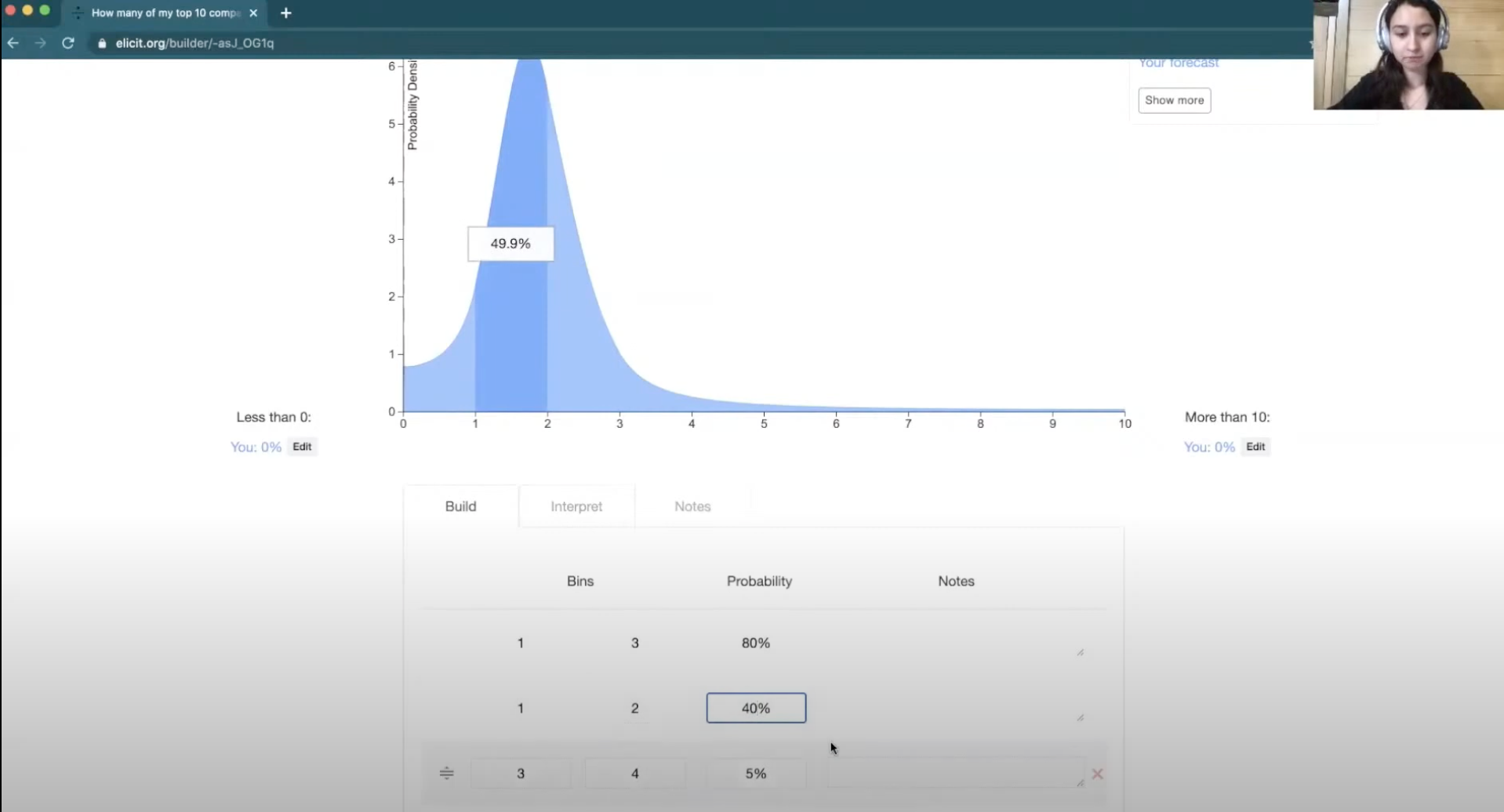
Then you can go back to “interpret”, and see that now it's two.
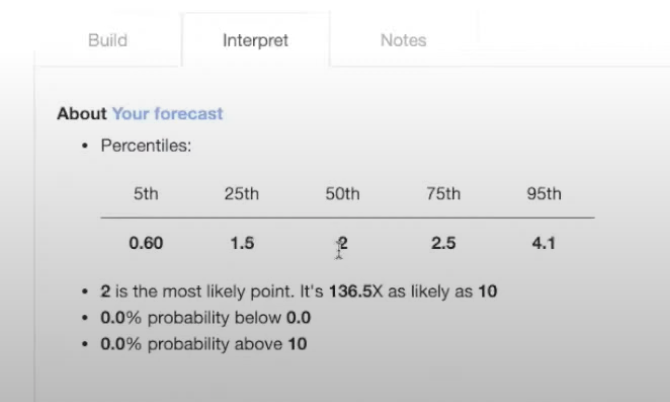
This seems a little more reasonable. You can save your snapshot again and it'll save your latest forecast.
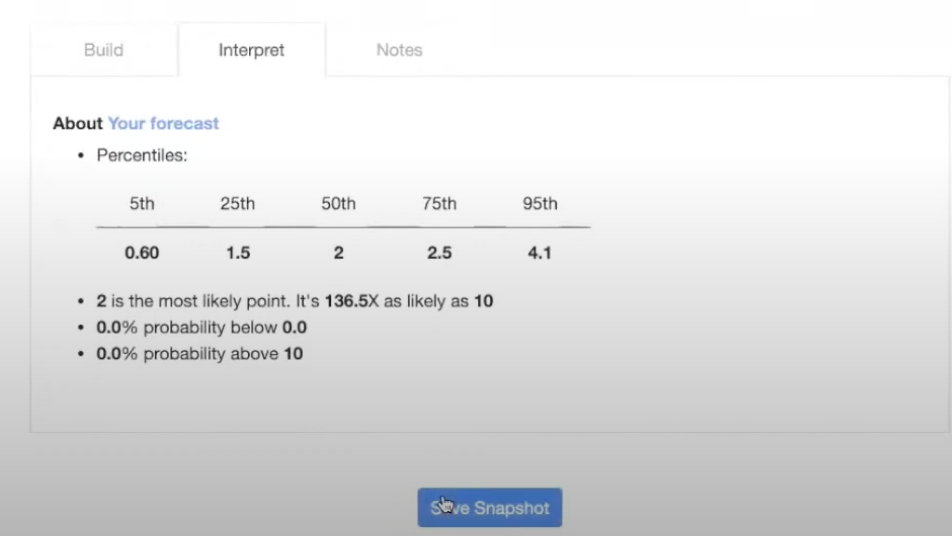
[00:14:15] You might want to compare this to what a friend says to see where you disagree. You can send them the snapshot URL.
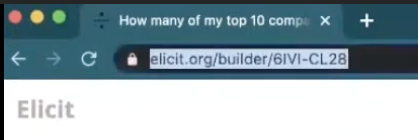
They can click on this title to get to a blank distribution so that they can make their own forecast.
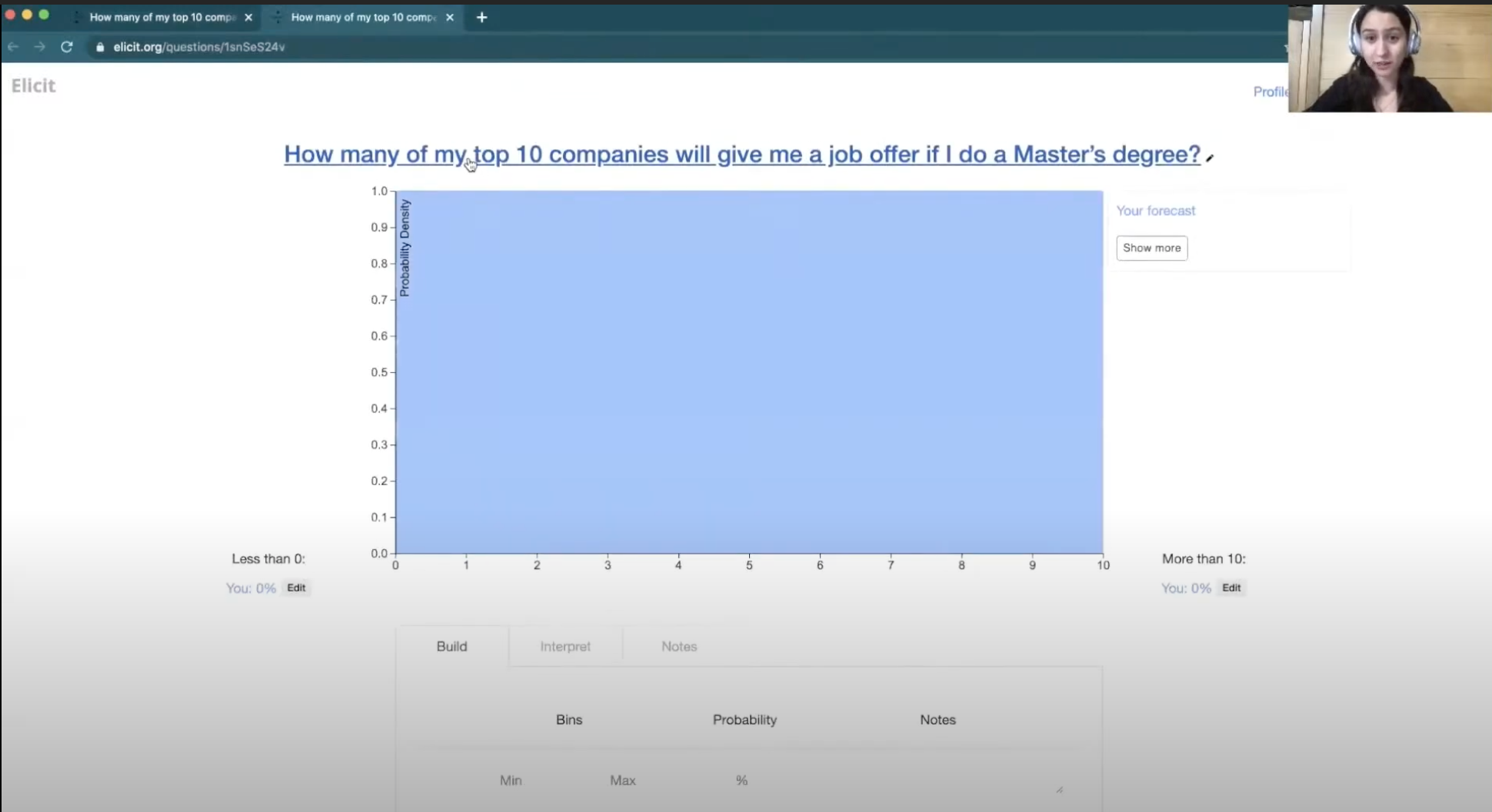
Maybe they're way more optimistic and they think it's probably between two and six. They save their forecast, send the URL back to you, and you can import it to compare.
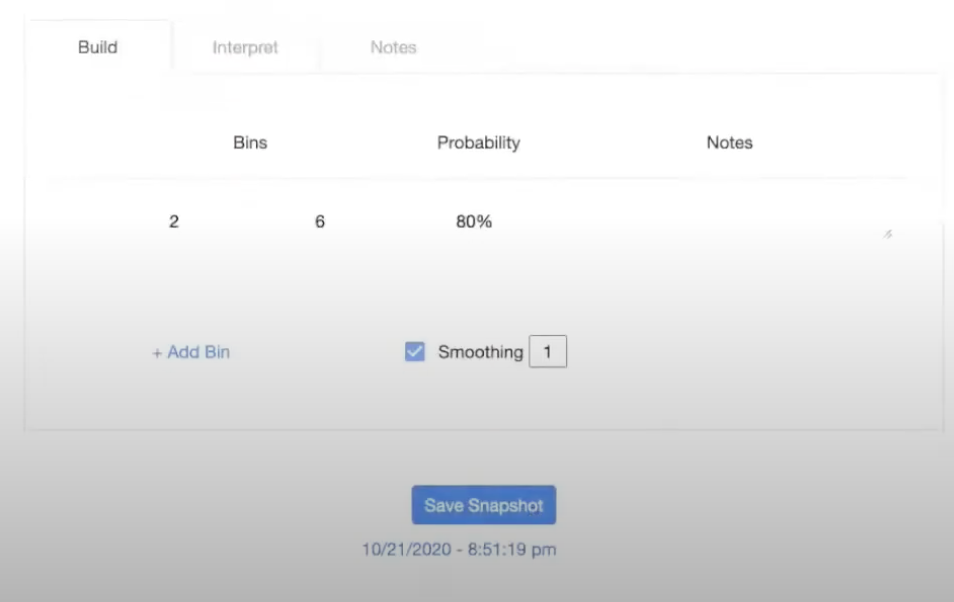
You can see the two forecasts here.
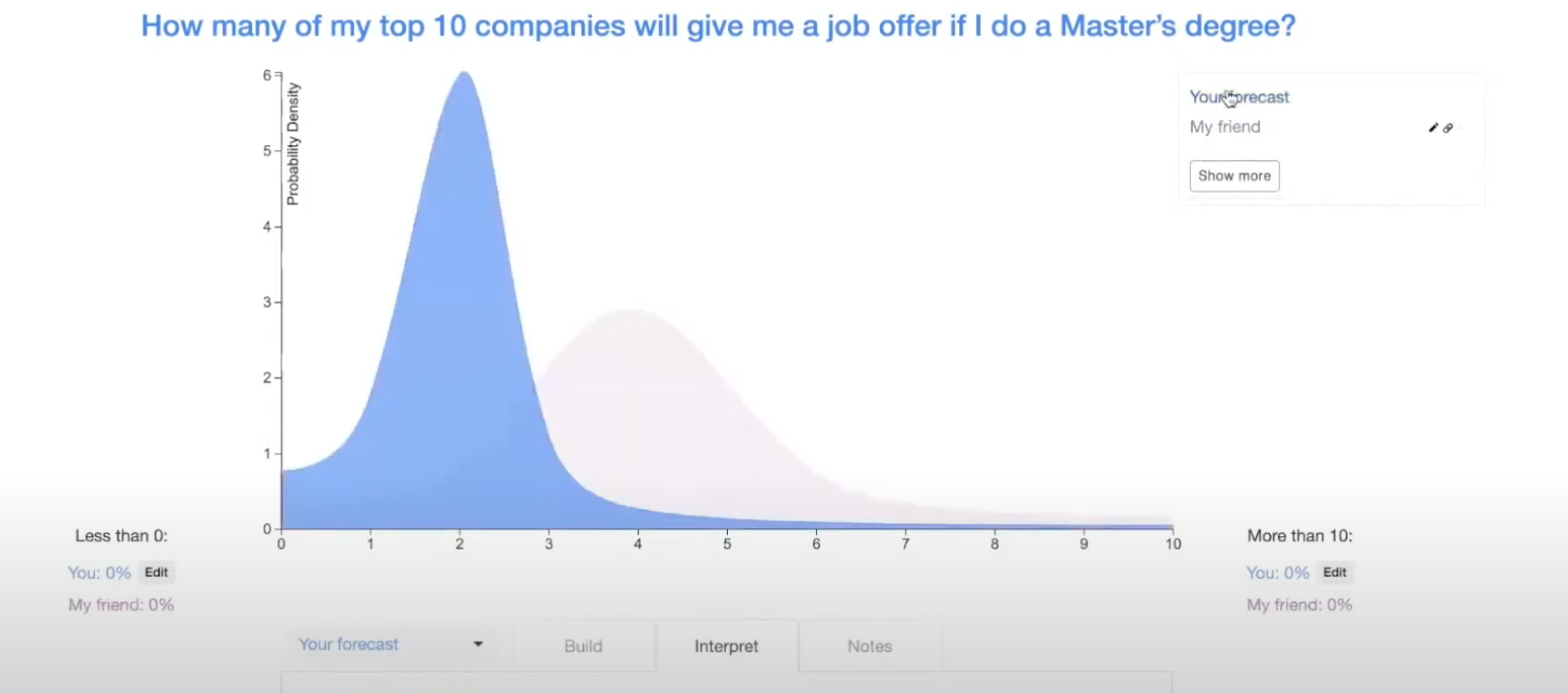
You'll notice in the “interpret” tab that there is now a section to compare your forecast with your friend's forecast, so it tells you where you disagree the most. It also gives you a possible bet. If you really believe your forecasts, you can enter into this bet.
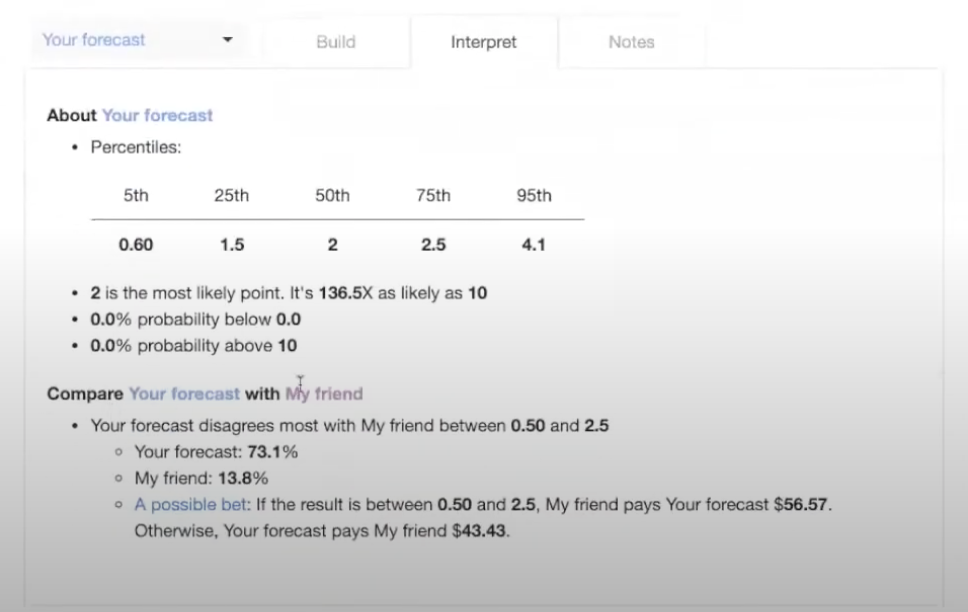
[00:15:03] What are some next steps you can take? The biggest thing is to just start forecasting. [...]
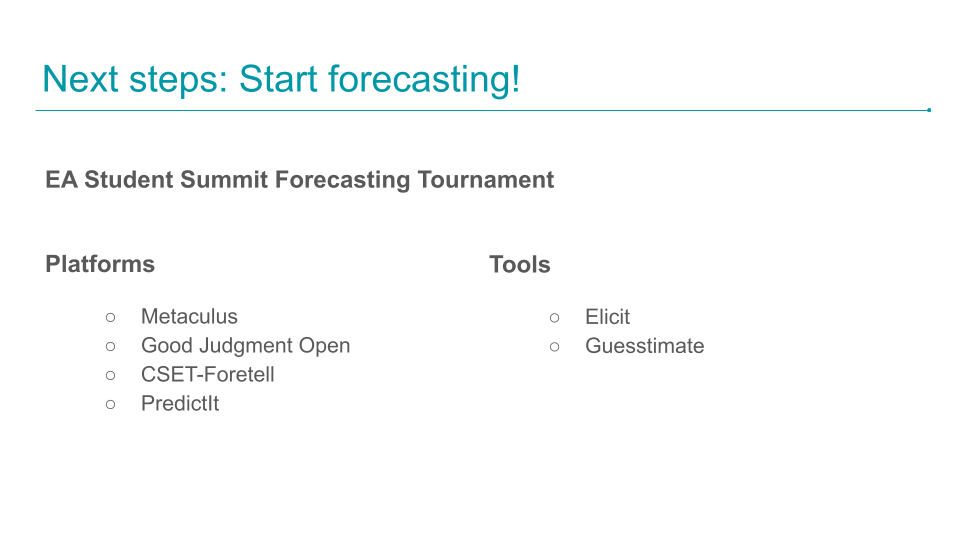
There are several platforms that have public questions that you can forecast on to start getting a track record:
There are also several prediction markets like PredictIt.
Then, there are a few tools that you can use to make your forecasts. So Elicit lets you make forecasts for continuous questions. For binary questions, you can also make forecasts that you can submit to Metaculus. And then Guesstimate is a tool built by Ozzie Gooen, and there is a link to a video that explains how to use Guesstimate, which is for building causal models of forecasts.
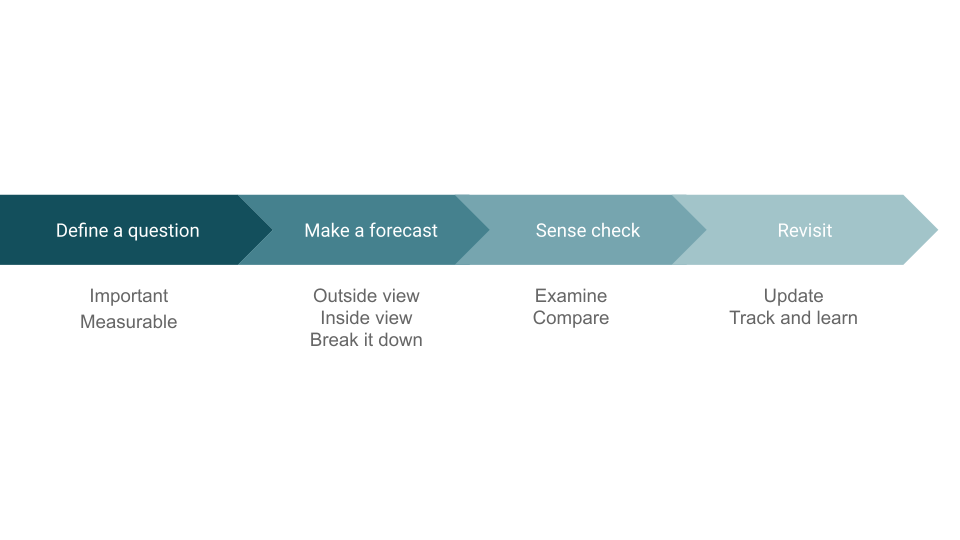
[00:16:05] Just to recap, today we talked about a process for forecasting:
- Define a question
- Make sure the question is important and measurable
- Make a forecast starting with the outside view
- Add the inside view
- Break the question down into sub-questions to make it easier
- Sense-check by examining the implications of your beliefs and comparing them to other people’s
- Revisit and update your forecasts to track them and learn from how they resolve
Thank you so much for listening. I'm excited to hear your questions, and you can also email any questions to amanda@ought.org.
Q&A
Habiba [00:16:44]: Thank you for that talk, Amanda. I can see that we've already had some questions come in, but feel free to keep asking them and we'll see how many we can get through in the next 10 minutes of the session.
We've definitely had some questions around both the successes and limitations of forecasting. Jumping straight in, let’s start with the successes. Do you have any examples, Amanda, of some of the real-world successes of forecasting?
Amanda [00:17:06]: Yeah, totally. First, the forecasting field is pretty nascent and there is not a lot of research. It's still growing as a field.
I think the biggest success to look at [is described in] Superforecasting. If you haven’t read it, I really recommend it. It basically showed that teams of expert forecasters were able to outperform domain experts, and that people were able to improve quickly over time with training. There are a lot of examples in that book that I recommend checking out.
I also mentioned the platform Metaculus. And Metaculus does a lot of crowd aggregation forecasts. They recently did a test in which people using Metaculus forecasted against COVID experts, and they found that Metaculus outperformed the experts. And there are other EA organizations, including one called EpiFor, doing COVID forecasting that you can look up as well.
I think the difficult thing is gauging how to know — you have to have a benchmark. So basically, these are some examples of how training in forecasting can help you do better than other experts.
Habiba [00:18:23]: I think you're probably about to say the thing I was about to ask. I was wondering if you had any examples within Ought and your own experience where you've felt that forecasting has helped?
Amanda [00:18:35]: Definitely. We do a lot of forecasting at Ought around project timelines with a number of users. We do it frequently for evaluating features (e.g., “If we build this feature, how many users will we get?”). We find that useful for the reasoning process itself. It also helps us calibrate our plans for marketing initiatives based on how much uncertainty people express in the forecasts that they send.
Habiba [00:19:07]: Super. Thanks for that. Switching into the limitations, do you have a general sense of what some of the main ones of forecasting are?
Amanda [00:19:16]: Definitely. The first is that forecasting is best in short-term situations where you have really quick feedback loops. There's not a lot of research into how well we forecast events that are really far into the future. It's very hard to do. In general, we're not calibrated to forecast events that are 100 years into the future, and that's a lot of what Ought is trying to work on. It's breaking those down into things that we can be better calibrated on and evaluate. That's one big limitation.
[00:19:46] There’s another one around expressing too much precision. There are a lot of things that we have great uncertainty on, and sometimes if you're trying to be really precise, forecasting can lead you down a pathway of being too confident in your numbers.
We can get into this in some of the later questions, but there are also obviously limitations around predicting so-called “black swan” events. How do you predict events that are really out of distribution? I think this is obviously a limitation of trying to forecast whether an event is going to occur, or what kinds of scenarios you might see. But I think there's still a lot of value in model-building and forecasting.
Habiba [00:20:40]: Yes. To pick up on that last point, people are curious about your take on Nassim Taleb's critique of forecasting. So I was wondering if you could summarize your understanding of what those “black swan” events are, and then give your take on that critique of forecasting?
Amanda [00:21:00]: I will say I'm not an expert on Taleb's work, but basically my interpretation of that question and the critique is that it's really hard to predict events that are extremely far out of distribution. Often with forecasting, we're talking about looking at past data and trying to predict new events based on what has already happened. And if you have really unpredictable events, what do you do with those? How do you predict them based on the past data?
I think the main way I would respond to this is by acknowledging that this is obviously a limitation. But I think there are two ways to think about forecasting. You can think of it as trying to get a really precise number that increases your confidence that an event is going to happen. Or you can think of it as a tool for helping you reason through what might cause events to happen. So, if you think about forecasting more as model-building, what are the different scenarios that could occur? What are the different causal things that would make those scenarios occur? What assumptions do we have? How do we figure out where we disagree with other people and have productive discussions about that?
I think that's a really important area of forecasting that is underrated if you only focus on the fact that we can’t predict Black Swan events, and therefore should give up.
Habiba [00:22:32]: Okay, thank you for that answer. That was very comprehensive. Thinking again about the kind of uses for forecasting, one of the questions is: “How, if at all, do you think prediction markets should be used in modern forecasting?”
Amanda [00:22:49]: Great question. Prediction markets are a little difficult because of the way they're regulated. I think some of the main ones are not based in the US. They're based elsewhere because people in the US can’t engage in these prediction markets.
I think the main way I think about these markets is in terms of whether they’re a useful crowd aggregation tool. You get the benefit of aggregating many different predictions. But there are limitations, particularly in the sense that there are often fees built in that go to the prediction platforms themselves, so you can't take the probabilities at face value because they're adjusting for those fees.
You also have to be really careful about how many people are actually participating and where they are in the world. I think it can be useful as one of your inputs in whatever model you're building, or however you're considering things, but I wouldn't take it at face value.
Habiba [00:23:47]: We've had a few questions come in from people talking about using Elicit and trying to build their own forecasts. When one questioner tries to use the Elicit app, they found it hard to have an intuitive feel for probability distributions. Do you have any advice on how they should try to approach constructing a distribution from their beliefs?
There’s also someone wondering how susceptible sense-checking probabilities is to bias, since you've already made a forecast quite robustly and then you're sort of undoing some of that careful thinking when you sense-check.
Amanda [00:24:26]: Great questions. On the first one, I think the intuitive feel is something that you build up over time. This is really where making a lot of predictions and learning to calibrate really helps. When you start out, you might not have a great sense of the difference between 30% and 50%. But the more you forecast, and the more feedback you get, you start to build that calibration muscle.
I find it useful to examine things from many different angles. Let’s say I think something is 30% likely. That means in three out of 10 worlds it’s going to occur. And then I like to think about those three worlds. Or sometimes you can just roll the dice, or choose a random number, and, based on how many times the random number pops up, see whether it seems reasonable. But I think the biggest thing is simply to do a lot of it and get quick feedback such that you can calibrate over time.
Habiba [00:25:21]: Yes, and I think I’d better stop you there. Sorry, I know I gave you a two-part question, but we’ve run out of time. [...]
Thank you so much, Amanda.