We summarize and compare several models and forecasts predicting when transformative AI will be developed.
Highlights
- The review includes quantitative models, including both outside and inside view, and judgment-based forecasts by (teams of) experts.
- While we do not necessarily endorse their conclusions, the inside-view model the Epoch team found most compelling is Ajeya Cotra’s “Forecasting TAI with biological anchors”, the best-rated outside-view model was Tom Davidson’s “Semi-informative priors over AI timelines”, and the best-rated judgment-based forecast was Samotsvety’s AGI Timelines Forecast.
- The inside-view models we reviewed predicted shorter timelines (e.g. bioanchors has a median of 2052) while the outside-view models predicted longer timelines (e.g. semi-informative priors has a median over 2100). The judgment-based forecasts are skewed towards agreement with the inside-view models, and are often more aggressive (e.g. Samotsvety assigned a median of 2043).
Introduction
Over the last few years, we have seen many attempts to quantitatively forecast the arrival of transformative and/or general Artificial Intelligence (TAI/AGI) using very different methodologies and assumptions. Keeping track of and assessing these models’ relative strengths can be daunting for a reader unfamiliar with the field. As such, the purpose of this review is to:
- Provide a relatively comprehensive source of influential timeline estimates, as well as brief overviews of the methodologies of various models, so readers can make an informed decision over which seem most compelling to them.
- Provide a concise summarization of each model/forecast distribution over arrival dates.
- Provide an aggregation of internal Epoch subjective weights over these models/forecasts. These weightings do not necessarily reflect team members’ “all-things-considered” timelines, rather they are aimed at providing a sense of our views on the relative trustworthiness of the models.
For aggregating internal weights, we split the timelines into “model-based” and “judgment-based” timelines. Model-based timelines are given by the output of an explicit model. In contrast, judgment-based timelines are either aggregates of group predictions on, e.g., prediction markets, or the timelines of some notable individuals. We decompose in this way as these two categories roughly correspond to “prior-forming” and “posterior-forming” predictions respectively.
In both cases, we elicit subjective probabilities from each Epoch team member reflective of:
- how likely they believe a model’s assumptions and methodology to be essentially accurate, and
- how likely it is that a given forecaster/aggregate of forecasters is well-calibrated on this problem,
respectively. Weights are normalized and linearly aggregated across the team to arrive at a summary probability. These numbers should not be interpreted too literally as exact credences, but rather a rough approximation of how the team views the “relative trustworthiness” of each model/forecast.
Caveats
- Not every model/report operationalizes AGI/TAI in the same way, and so aggregated timelines should be taken with an extra pinch of salt, given that they forecast slightly different things.
- Not every model and forecast included below yields explicit predictions for the snapshots (in terms of CDF by year and quantiles) which we summarize below. In these cases, we have done our best to interpolate based on explicit data-points given.
- We have included models and forecasts that were explained in more detail and lent themselves easily to a probabilistic summary. This means we do not cover less explained forecasts like Daniel Kokotajlo’s and influential pieces of work without explicit forecasts such as David Roodman’s Modelling the Human Trajectory.
Results
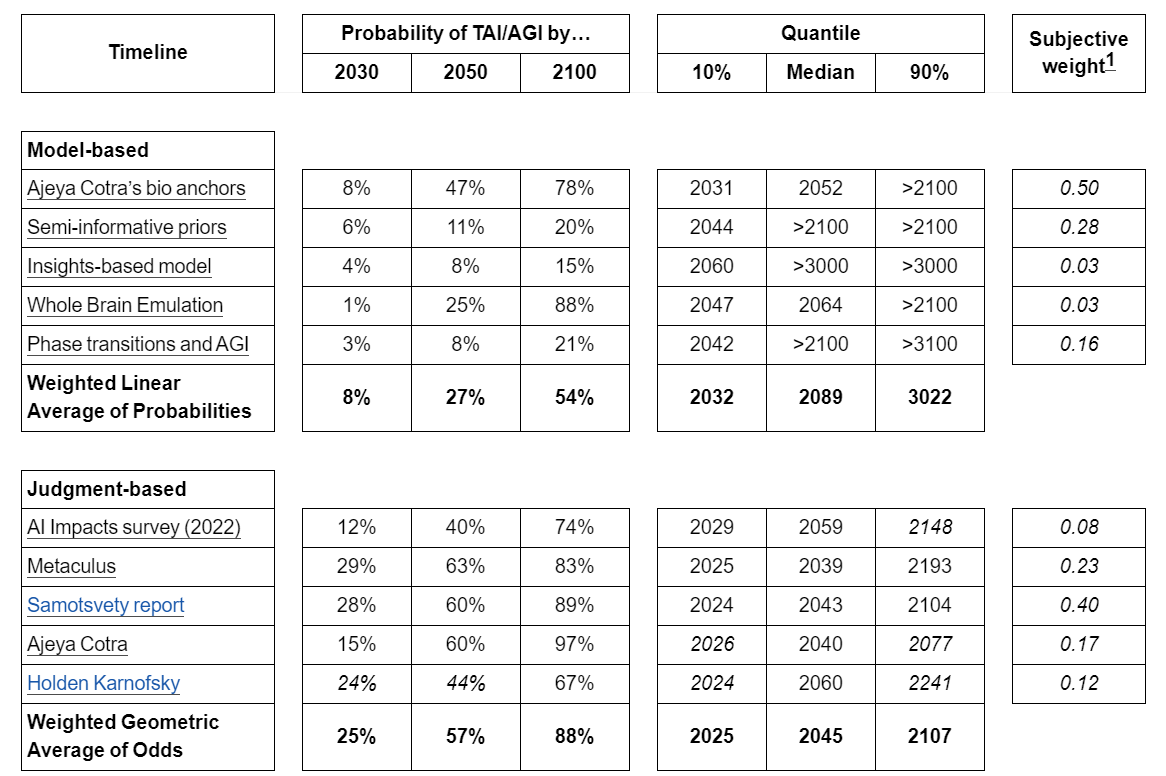
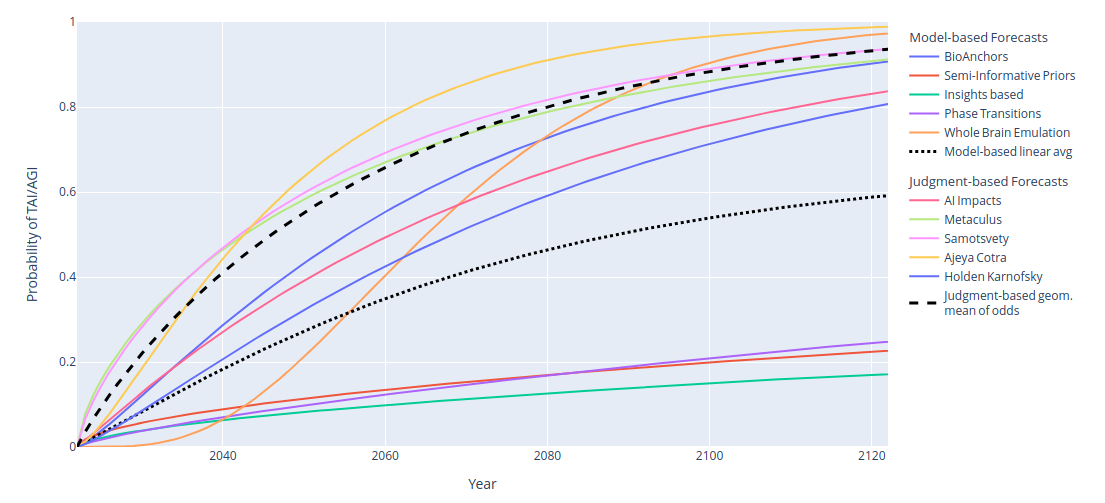
Some excellent content on AI timelines and takeoff scenarios has come out recently:
I'm curating this post, but encourage people to look at the others if they're interested.
Things I really appreciate about this post:
Other notes:
Thank you Lizka, this is really good feedback.
This seems pretty neat, kudos for organizing all of this!
I haven't read through the entire report. Is there any extrapolation based on market data or outreach? I see arguments about market actors not seeing to have close timelines, as the main argument that timelines are at least 30+ years out.
Extracting a full probability distribution from eg real interest rates requires multiple assumptions about eg GDP growth rates after TAI, so AFAIK nobody has done that exercise.
Yea, I assume the full version is impossible. But maybe there are at least some simpler statements that can be inferred? Like, "<10% of transformative AI by 2030."
I'd be really curious to get a better read on what market specialists around this area (maybe select hedge fund teams around tech disruption?) would think.
I don't think it's impossible - you could start from Harperin's et al basic setup [1] and plug in some numbers about p doom, the long rate growth rate etc and get a market opinion.
I would also be interested in seeing the analysis of hedge fund experts and others. In our cursory lit review we didn't come across any which was readily quantifiable (would love to learn if there is one!).
[1] https://forum.effectivealtruism.org/posts/8c7LycgtkypkgYjZx/agi-and-the-emh-markets-are-not-expecting-aligned-or
I notice that some of these forecasts imply different paths to TAI than others (most obviously, WBE assumes a different path than the others). In that case, does taking a linear average make sense? Consider if you think WBE is likely moderately far away, versus other paths are more uncertain and may be very near or very far. In that case, a constant weight on the WBE probability wouldn't match your actual views.
I am not sure I follow 100%: is your point that the WBE path is disjunctive from others?
Note that many of the other models are implicitly considering WBE, eg the outside view models.
Yeah, my point is that it's (basically) disjunctive.
Thanks, this is just great!
The medians for the model-based and judgement-based timelines are 2089 and 2045 (whose mean is 2067). These are 44 years apart, so I wonder whether you thought about how much weight to give to each type of model.